Molecular Chemistry Megatrend at G7 Summit: IBM's Leap in Quantum Centric Super Computing
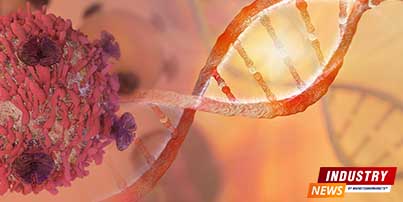
This News Covers
- The Data Dependency of AI in Chemistry
- The Intersection of AI and Biomedical Sciences
- How IBM is readying a series of large language models for molecular chemistry?
- The Impact Generative AI on Top Molecular Chemistry Companies
- What are the policies that should govern use of LLMs and AI in molecular chemistry?
- Exploring the Confluence of Chemistry and Artificial Intelligence
- Which top subdomains within chemistry use AI and large language models?
IBM has announced a 10-year, $100 million partnership with the University of Tokyo and the University of Chicago to pioneer a quantum-centric supercomputer with an unprecedented 100,000 qubits. This groundbreaking initiative heralds a new era in high-performance computing, potentially enabling solutions for global challenges that existing supercomputers can't tackle. Such a powerful system could revolutionize understanding of chemical reactions and molecular dynamics, leading to advancements like more efficient methods for carbon capture, sustainable materials for electric batteries, and energy-efficient fertilizers.
MarketsandMarkets welcomes this development and our editors share their views.
The Data Dependency of AI in Chemistry
The deployment of artificial intelligence (AI) in the field of chemistry, particularly for the development of generative tools, depends heavily on the availability and accessibility of reliable, bias-free training datasets. These datasets need to be expansive, featuring both experimental and simulated data including historical records and information from unsuccessful experiments. Although machine learning promises a revolution in chemical research and substance synthesis, the lack of data currently available is preventing AI from reaching its full potential.
The Role of AI in Retrosynthesis and Inverse Design
AI tools have been developed for conducting retrosynthesis and inverse design in chemistry. Retrosynthesis AI, like the 3N-MCTS system, involves determining the best starting materials and reaction sequence to create a desired chemical structure. Meanwhile, inverse design starts with desired physical properties, then identifies substances that exhibit these properties. Both applications depend heavily on the AI system's knowledge of specific chemical structures and their reactions. While these AI applications have shown promise, their adoption remains low due to the insufficient comprehensive knowledge in these systems, often due to incomplete published reaction results.
The Success Story of AlphaFold and the Need for More Data
AlphaFold, a protein-structure-prediction tool, stands as an exemplar of the potential of AI in chemistry, having been successfully trained on a robust dataset of over 200,000 structures from the Protein Data Bank. This demonstrates the transformative power AI can have when given sufficient high-quality data. However, the availability of such extensive datasets is still lacking in most areas of chemistry. Efforts are underway to extract data from research papers and existing databases, automate laboratory systems, and use both real and simulated data for training.
The Demand for Open Data and Standardized Recording
AI tools require access to open data to reach their full potential. The publishing culture in chemistry must evolve to accommodate this, with a shift towards data deposition in open repositories. Furthermore, a consistent format for data recording is crucial, along with the inclusion of negative outcomes or failed experiments. The generation of comprehensive, diverse and accessible datasets can help transform AI from being merely an over-hyped technology to a practical tool that can revolutionize chemistry.
The Intersection of AI and Biomedical Sciences
The potential of AI extends beyond the field of chemistry and into biomedical sciences. Discussed in a panel by Northwestern University, advancements in AI are identified as a "hot topic" in improving the quality of human life. This includes advances in the use of AI for studying aging, eradicating tumors, and developing precision medicine. The panel emphasized the importance of integrating disciplines, cross-collaboration and increasing diversity in the sciences to fully realize the potential of AI. The melding of AI with other scientific fields underscores the technology's profound ability to catalyze innovation across a spectrum of research.
How IBM is readying a series of large language models for molecular chemistry?
IBM's focus on generative AI and hybrid cloud signifies a critical inflection point in the AI field. Through the deployment of foundation models, particularly in molecular chemistry, IBM is paving the way for transformative changes in scientific and healthcare domains. The economic implications are profound, and policymakers will need to ensure these technological advancements result in broad-based benefits.
IBM's venture into generative AI and hybrid cloud, particularly in the area of foundation models for molecular chemistry, represents a critical progression in the development and deployment of AI applications in the field of science and healthcare. This leap comes at a time when many organizations are only beginning to explore machine learning applications, signaling IBM's determination to be at the forefront of technological innovation.
The primary shift in IBM's approach is from creating AI applications in isolation to adopting a foundation model strategy. This strategic change in approach has been spearheaded by advancements in AI models such as GPT by OpenAI, which are capable of ingesting vast volumes of data to generate complex, sophisticated results. IBM's decision to utilize foundation models aims to allow organizations to begin with a robust, pre-trained model and customize it to suit their specific needs. This is a pragmatic approach that relieves enterprises from the arduous task of developing complex models from scratch, reducing the entry barrier to advanced AI utilization.
IBM's emphasis on foundation models in molecular chemistry has vital implications. This approach could significantly expedite drug discovery processes, given that the models would likely be trained on vast chemical databases, allowing for efficient generation of possible molecular structures and interactions. This could transform the field of molecular chemistry, enabling faster, more effective responses to health crises, such as pandemics.
Watsonx, IBM's suite of AI-based applications and tools focusing on AI model lifecycle management, model governance, and a new data lakehouse, is an essential element of their strategy. Watsonx includes "classical" capital-W Watson tools, reinforcing IBM's commitment to machine learning and neural networks, while also allowing a new generation of AI to flourish within the corporation.
One of the principal challenges for enterprises will be choosing and navigating the correct foundation model. IBM plans to guide this selection process, providing specific models for certain use cases. In the long term, generative AI could assist organizations in this model selection process, which could eventually lead to AI systems helping to customize themselves, a reflection of a profound shift in AI utilization.
The development of Watsonx, as a platform for AI models' lifecycle management and governance, is a critical part of IBM's strategy. Incorporating classical Watson tools such as Watson Orchestrate, Watson Assistant, and Watson Discovery, Watsonx will support models from the open-source community, underscoring IBM's commitment to collaborative and transparent AI development.
IBM's data strategy, centered on watsonx.data, a new data lakehouse based on Apache Iceberg, will ensure that the foundation models have access to vast amounts of data. This implementation stands out with remote distributed caching, enabling data distribution across multiple physical instances, and supporting hybrid cloud deployment.
From the perspective of a world-leading economist, IBM's recent strategic direction could have far-reaching implications. On the one hand, it exemplifies the private sector's pivotal role in technological innovation, propelling advancements that can benefit broader society. IBM's work could potentially contribute to healthcare efficiency, reducing costs and improving outcomes, which are key factors in any country's economic health.
On the other hand, this shift could also exacerbate the digital divide, especially for firms and countries lacking the resources to access and implement advanced AI technology. Policymakers must therefore strive for inclusive growth, ensuring that the benefits of AI and other technologies are broadly shared.
The Impact Generative AI on Top Molecular Chemistry Companies
These companies, along with others operating in the molecular chemistry space, could gain a significant advantage by leveraging IBM's generative AI. Not only could this lead to more efficient research and development processes, but it may also result in the discovery of groundbreaking solutions to many of today's most pressing health and environmental challenges. Here are the top five companies in the molecular chemistry field that could be influenced by this development:
- Pfizer Inc. (New York City, USA) - Pfizer is a global pharmaceutical corporation and one of the world's largest drug manufacturers. Pfizer's key offerings include medicines, vaccines, and many health-related consumer products. Pfizer is publicly listed and does not raise funds through venture capital or private equity. This development could allow Pfizer to expedite its drug discovery process and develop even more effective health solutions.
- BASF SE (Ludwigshafen, Germany) - BASF is the world's largest chemical producer, offering a wide range of products from chemicals, plastics, performance products, crop technology, and oil and gas. Being a publicly listed company, it doesn't typically raise funds like startups. IBM's generative AI could help BASF streamline its chemical production process and discover new compounds more efficiently.
- Dow Inc. (Midland, USA) - Dow is a multinational chemical corporation focused on science and technology, delivering innovative solutions across packaging, infrastructure, and consumer care. Similar to Pfizer and BASF, Dow is publicly listed. Dow could use IBM's AI technology to optimize its manufacturing processes, creating more sustainable and efficient chemical solutions.
- GlaxoSmithKline plc (Brentford, UK) - GSK is a leading global healthcare company specializing in pharmaceuticals, vaccines, and consumer healthcare. It is publicly listed, hence, doesn't typically raise venture capital. GSK could leverage IBM's AI technology to improve its pharmaceutical and vaccine research, potentially leading to the quicker development of new drugs and treatments.
- Novartis International AG (Basel, Switzerland) - Novartis is a multinational pharmaceutical company offering healthcare solutions that address the evolving needs of patients worldwide. Like the others, Novartis is publicly traded. With IBM's new AI models, Novartis could enhance its drug discovery and research process, potentially revolutionizing its approach to healthcare.
What are the policies that should govern use of LLMs and AI in molecular chemistry?
The unveiling of IBM's new generative AI models and hybrid cloud strategy could potentially revolutionize molecular chemistry-related businesses. The enhanced computation and predictive capabilities could allow these businesses to streamline their research and development efforts, optimizing processes and significantly reducing time-to-market for innovative solutions.
As the advent of artificial intelligence (AI) and large language models (LLMs) has greatly transformed the molecular chemistry domain, it's crucial to enforce stringent policies that ensure their responsible and ethical use. The integration of these advanced tools, referred to as foundation models, promises immense benefits including rapid molecule structure prediction and improved medicinal designs. Nevertheless, potential risks like bias, content hallucination, and unforeseen harm also loom large, warranting a proactive stance from policymakers.
Firstly, promoting transparency is paramount. Clear guidelines should be established detailing the data used for training these models, the intended applications, potential risks, and mitigation strategies. This would engender trust and allow users to make informed decisions.
Secondly, flexible approaches should be employed to address the dynamic nature of AI technology. Rather than rigid, one-size-fits-all policies, adaptable frameworks that can evolve with the technology should be favoured.
Thirdly, the policies should differentiate between various business models, considering the scope and potential impact of the AI applications. Industries like pharmaceuticals, which could have profound public health implications, may require stricter regulation compared to others.
Finally, emerging risks must be carefully studied. Policies should be adaptive to address these evolving threats, ensuring the ongoing safety of users and society at large.
As foundation models continue to revolutionize molecular chemistry, a balanced, risk-based approach to regulation should be adopted. This way, we can harness the technology's potential benefits while effectively mitigating its inherent risks.
Exploring the Confluence of Chemistry and Artificial Intelligence
Chemistry and artificial intelligence (AI) interact in a myriad of intriguing ways. Artificial intelligence, especially machine learning algorithms, are employed to decode complex chemical reactions and predict molecular behaviors. Through processing vast datasets and identifying patterns, AI can enable scientists to expediently understand and predict the properties of various chemical compounds, thereby accelerating the pace of chemical research and discovery.
The Emergence of AI as a Solution Seeker in Chemical Conundrums
Indeed, there are AI models specifically developed to tackle intricate problems in chemistry. For instance, models like IBM's generative AI are utilized for drug discovery, which involves understanding the complex interactions between chemical compounds and biological systems. These AI models can simulate these interactions and forecast their outcomes, thus aiding in the development of new pharmaceutical compounds.
Capitalizing on AI's Strengths in the Realm of Chemistry
AI provides several notable benefits in the field of chemistry. First, it enhances efficiency by enabling high-throughput screening and rapid prediction of compound properties. Second, AI promotes innovation by discovering new chemical structures, reactions, and materials, which may be overlooked by traditional research methodologies. Lastly, AI can reduce the cost and time associated with experimental trials by predicting the success of a reaction before it is physically performed, thereby revolutionizing the field of chemistry with its potent capabilities.
Which top subdomains within chemistry use AI and large language models?
AI and large language models find application in several sub-domains within chemistry, including:
- Medicinal and Pharmaceutical Chemistry: AI models are used to predict the properties of potential drugs, including their bioactivity, toxicity, and pharmacokinetics. AI tools can also simulate interactions with biological targets, greatly aiding the drug discovery and development process.
- Materials Chemistry: AI is used to predict the properties of novel materials before they are synthesized, saving substantial time and resources. This has applications in fields as diverse as semiconductors, battery technologies, and nano-engineering.
- Organic and Inorganic Chemistry: AI models can be trained on databases of known chemical reactions to predict the outcomes of novel reactions. This assists in planning synthetic routes and understanding mechanisms of reactions.
- Analytical Chemistry: AI can help analyze complex data from instruments like mass spectrometers, NMR spectrometers, and chromatography systems, enabling more accurate and efficient identification and quantification of chemical substances.
- Chemoinformatics: Large language models can be used to extract and structure chemical information from text, such as scientific articles and patents. This is particularly useful in systematically gathering and analyzing data for drug discovery and materials design.
- Environmental Chemistry: AI can help predict the environmental impact of certain chemicals, their biodegradability, and their potential effects on ecosystems. It can also be used to develop and optimize methods for water treatment, air purification, and waste management.
By harnessing AI and large language models in these domains, we can accelerate scientific progress and make chemistry more efficient, predictive, and sustainable.
References
- IBM Launches $100 Million Partnership with Global Universities to Develop Novel Technologies Towards a 100,000-Qubit Quantum-Centric Supercomputer
- IBM Doubles Down On Generative AI And Hybrid Cloud
- For Chemists, The AI Revolution Has Yet To Happen
- Artificial Intelligence In Chemistry: Current Trends And Future Directions
- AI for chemistry
- Large Language Models Could Be The Catalyst For A New Era Of Chemistry
GET AHEAD
Top Research Reports to Fuel Your Industry KnowledgeEditor's Pick
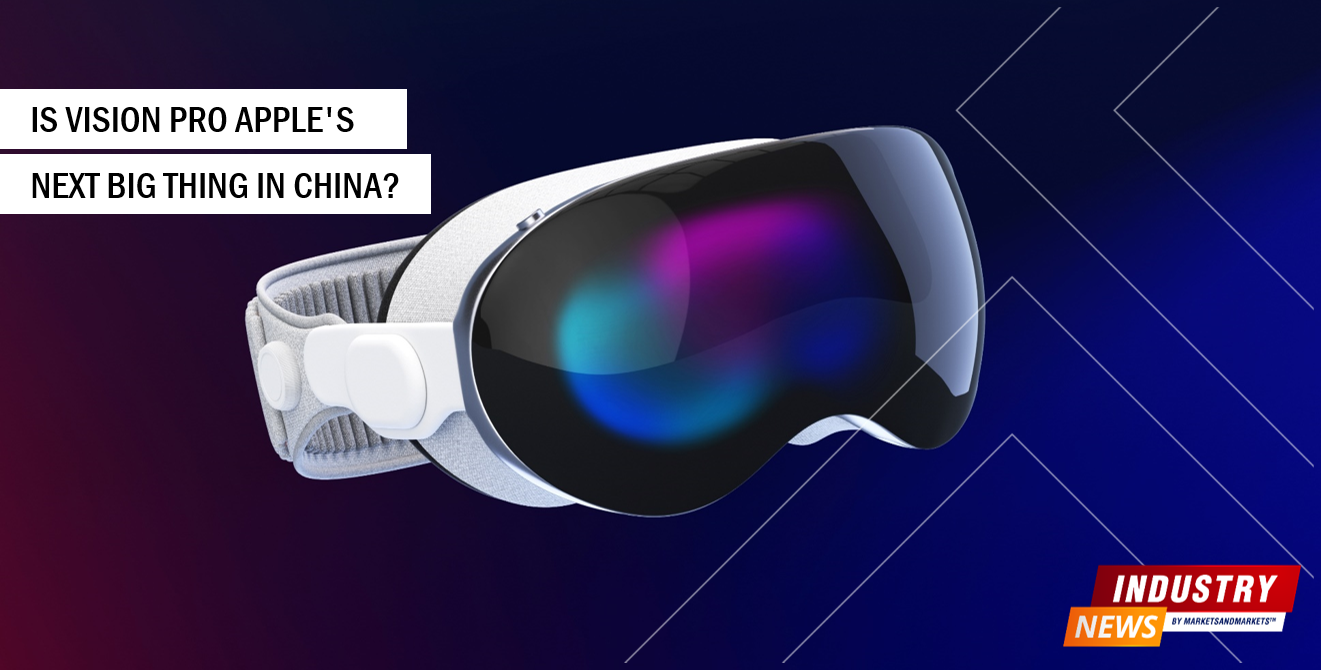
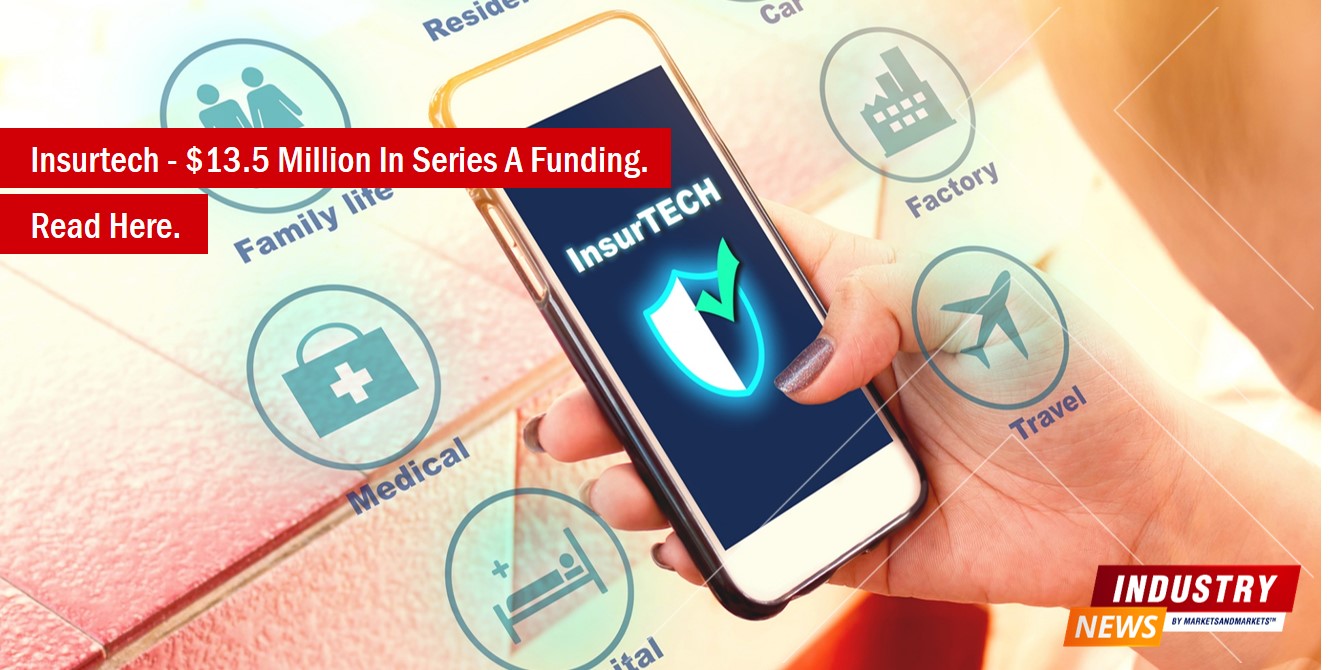
Information and Communication Technology
Insurtech Funding News - Coverdash raises USD 13.5 Million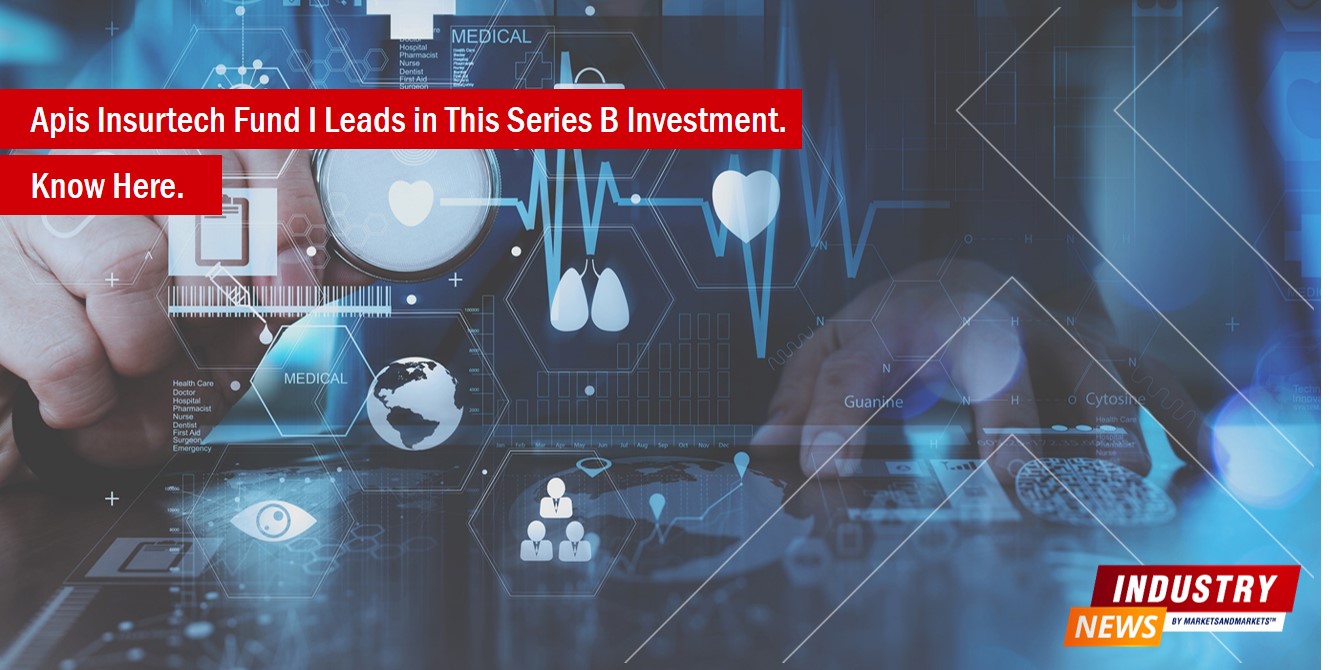
PODCASTS
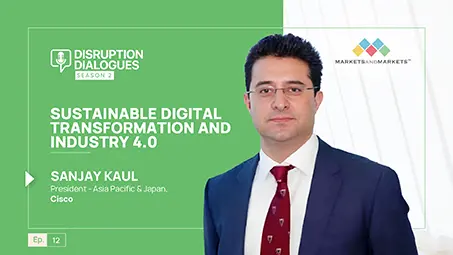
Sustainable Digital Transformation & Industry 4.0
Sanjay Kaul, President-Asia Pacific & Japan, Cisco, and host Aashish Mehra, Chief Research Officer, MarketsandMarkets, in conversation on unraveling 'Sustainable Digital Transformation and Industry 4.0'
11 July 2023|S2E12|Listen Now
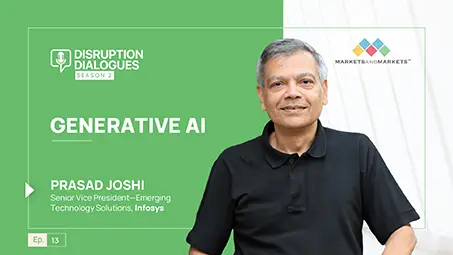
Generative AI
Prasad Joshi, Senior Vice President-Emerging Technology Solutions, Infosys, and host, Vinod Chikkareddy, CCO, MarketsandMarkets, in exploring the recent advances in AI and the generative AI space.
7 Nov 2023|S2E13|Listen Now
Molecular Biology Enzymes


Download Whitepaper
IBM's focus on generative AI and hybrid cloud signifies a critical inflection point in the AI field.
AI provides several notable benefits in the field of chemistry.
