Top Biotech Growth Factors in 2023 - Companies, Researchers, Future
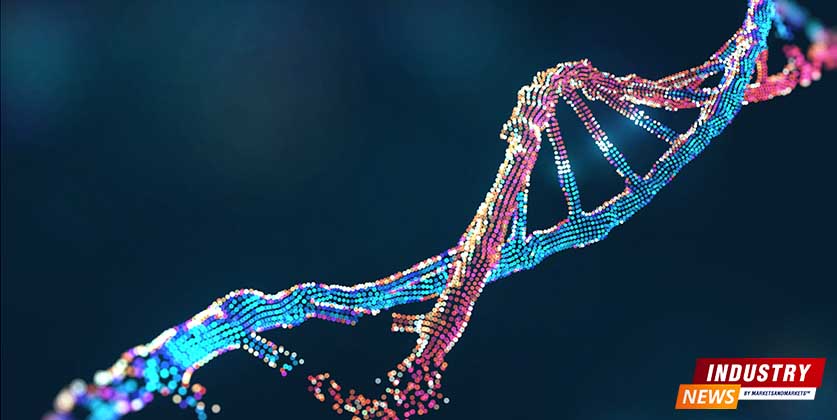
This News Covers
- What is generative AI in biotechnology?
- How are NVIDIA, Mayo Clinic and Google using Generative AI in biotechnology?
- How is AI used in biotechnology?
- Recent developments in Convolutional Neural Networks in Biotechnology?
- Which biotech companies are using AI?
- What is the current state of biotech investments in AI in the United States?
- Why Investors are keenly interested in AI powered Biotech?
- Impact of AI on these domains of biotech: neurodegenerative diseases, immuno-oncology, cardiovascular diseases, metabolic diseases?
- What is the CAGR for the AI in Drug Discovery Market in Japan and South Korea?
- What is the CAGR for the AI in Drug Discovery Market in the USA?
- How does generative AI in biology contribute to the discovery and design of novel molecules and compounds?
- What are the applications of generative AI in drug discovery and development processes in biotechnology?
- How is generative AI utilized in the field of synthetic biology to design and engineer new biological systems?
- In what ways does generative AI support protein folding prediction and structure determination in biotechnology research?
- What future developments and trends can be anticipated in the application of generative AI in biology and biotechnology?
What is generative AI in biotechnology?
Generative AI has emerged as a transformative tool in biotechnology, opening up new avenues for research, development, and application. The technology's ability to generate novel, high-quality data and simulate complex biological systems has proven particularly valuable in this field.
It is reshaping biotechnology.
MarketsandMarkets welcomes this development and our editors share their views.
How are NVIDIA, Mayo Clinic and Google using Generative AI in biotechnology?
Accelerating drug discovery, enabling personalized medicine, and enhancing our understanding of biological structures are just some of the realised advancements and are no longer science fiction. As AI continues to evolve, its application in biotechnology is expected to yield more groundbreaking developments, transforming healthcare as we know it.
NVIDIA, a leading player in AI and computing, has made significant advancements in leveraging generative AI for drug discovery. Their platform, Clara Discovery, integrates AI, simulation, and visualization to accelerate the discovery of life-saving drugs. The platform's generative models can predict the properties of potential drug candidates, significantly reducing the time and cost of traditional drug discovery processes. NVIDIA's GANs (Generative Adversarial Networks) have also been used to generate synthetic patient data, enabling researchers to conduct extensive testing without compromising patient privacy.
Mayo Clinic, a renowned healthcare organization, has collaborated with the UK-based AI firm, BenevolentAI, to apply generative AI in clinical research. The partnership aims to use AI to create advanced, disease-specific models that can predict disease progression and response to therapy. This collaboration is a prime example of how generative AI can be used to personalize treatment plans and improve patient outcomes.
Google's DeepMind has also made strides in the healthcare sector with its AI system, AlphaFold. This AI tool uses generative models to predict protein structures with remarkable accuracy. Understanding protein structures is crucial in biotechnology as it can lead to breakthroughs in disease understanding and drug discovery. AlphaFold's success in the 2020 Critical Assessment of protein Structure Prediction (CASP) competition underscores the potential of generative AI in revolutionizing biotechnology.
How is AI used in biotechnology?
Use of artificial intelligence (AI) in the field of biotechnology. AI is becoming increasingly prevalent in the life sciences, with applications ranging from machine learning and big data analytics to knowledge discovery and data mining, biomedical ontologies, knowledge-based reasoning, natural language processing, decision support, and reasoning under uncertainty.
When advances in biotechnology are combined with advances in AI, new potential solutions become available that can help address many global problems and contribute to important sustainability development goals. These include food security, health and wellbeing, clean water, clean energy, responsible consumption and production, climate action, life below water, and the sustainable use of terrestrial ecosystems.
AI technologies such as machine learning, deep learning, and explainable AI (XAI) are being used to transform biotechnology. For instance, AI can be used to analyze large datasets, make predictions, and even generate new hypotheses in biotechnology research. This can lead to more efficient and effective drug discovery, disease diagnosis, and other biotechnological applications.
The use of specific AI technologies that are being used in various ways to advance the field of biotechnology such as:
- Generative pretrained transformer (ChatGPT)
- convolutional neural networks (CNN)
- conditional random fields (CRF)
- cyberphysical systems (CPS)
- domain specific language (DSL)
- graph neural network (GNN)
- long short-term memory (LSTM)
- natural language processing (NLP)
- probabilistic graphical model (PGM), and
- reinforcement learning (RL).
Recent developments in Convolutional Neural Networks in Biotechnology?
In 2023, several advancements and research initiatives have been made in the field of convolutional neural networks (CNNs) specifically in biotechnology. Here are some highlights:
- A study published in [Frontiers] discusses the use of a Multi-Mode Convolutional Neural Network to reconstruct time series of satellite-derived chlorophyll-a concentration, a proxy of phytoplankton biomass. This research could have significant implications for understanding marine biology and climate change.
- An article in [Nature] explores the use of molecular convolutional neural networks with DNA regulatory mechanisms. This could potentially revolutionize the way we understand and manipulate genetic information.
- A [research paper] on the National Center for Biotechnology Information (NCBI) discusses how CNNs have been used to extract information from various datasets of different dimensions. This approach has led to accurate interpretations in several subfields of biological research, like pharmacogenomics.
- [Phys.org] provides updates on the latest developments in CNNs, including their application in lensless complex amplitude demodulation based on deep learning in holographic data storage.
- An article on [TechXplore] discusses how photonics is revolutionizing CNNs. Integrated photonic convolutional neural networks are being used to speed up calculations in CNNs using special optical devices.
These advancements underscore the growing importance of CNNs in biotechnology, with potential applications ranging from genetic research to data analysis and beyond.
Which biotech companies are using AI?
- Deep Genomics: Deep Genomics combines genomics with AI to develop novel therapeutics. Their platform analyzes genomic data to identify genetic variants associated with diseases and predict their impact on biological processes.
- BenchSci: BenchSci uses AI to help scientists in the life sciences discover and select the most suitable antibodies for their experiments. Their platform analyzes published scientific papers and extracts relevant antibody data, improving the efficiency and reliability of antibody selection.
- Recursion Pharmaceuticals: Recursion Pharmaceuticals uses AI and machine learning to identify new treatments for rare genetic diseases. Their platform screens thousands of potential drugs in various disease models to identify promising candidates for further development.
- Atomwise: Atomwise employs AI to accelerate drug discovery by simulating and predicting the interactions between small molecules and target proteins. Their platform enables virtual screening of millions of compounds, aiding in the identification of potential drug candidates.
- Insitro: Insitro integrates AI and machine learning with high-throughput biology to develop precision medicines. Their platform analyzes large-scale datasets to understand disease biology and identify novel targets for therapeutic intervention.
- BERG: BERG applies AI and data analytics to discover and develop drugs across various therapeutic areas. Their platform integrates patient data, biological samples, and clinical information to identify disease biomarkers and guide drug discovery.
- PathAI: PathAI uses AI and machine learning to improve pathology and pathology research. Their platform analyzes digitized pathology images to assist pathologists in diagnosing diseases and predicting patient outcomes.
Biotech companies that are harnessing the power of AI. The field of AI in biotech is rapidly evolving, and many other companies are incorporating AI into their research and development processes to drive innovation and improve patient outcomes.
What is the current state of biotech investments in AI in the United States?
Growing importance of AI in biotech and provides insights into the investments in AI within the biotech sector in the US. Here are some key points:
- Increasing Investments: Biotech companies are increasingly investing in AI technologies to drive innovation, improve efficiency, and accelerate drug discovery and development processes. The investments in AI within the biotech sector have been on the rise.
- AI Adoption: Biotech companies are adopting AI across various areas, including drug discovery, clinical trials, precision medicine, and manufacturing. AI is being used to analyze large datasets, predict drug efficacy, identify biomarkers, optimize clinical trial design, and improve manufacturing processes.
- Partnerships and Collaborations: Biotech companies are partnering with AI technology providers, academic institutions, and other organizations to leverage AI capabilities. These collaborations aim to combine biotech expertise with AI capabilities to achieve breakthroughs in research and development.
- Startups and Venture Capital: The article highlights the increasing number of startups focused on AI in biotech. These startups are attracting significant venture capital investments, indicating the growing interest in AI-driven biotech innovations.
- Regulatory Considerations: The integration of AI into biotech raises regulatory considerations. Regulators are working to establish frameworks and guidelines for AI-driven technologies in the biotech sector to ensure patient safety, data privacy, and ethical use of AI.
Why Investors are keenly interested in AI powered Biotech?
Here are some key reasons:
- Accelerating Drug Discovery and Development: AI has the potential to significantly accelerate the drug discovery and development process by analyzing large datasets, predicting drug efficacy, and identifying potential candidates for further research. This can save time, reduce costs, and increase the efficiency of bringing new drugs to market.
- Precision Medicine and Personalized Treatments: AI enables personalized medicine by analyzing individual patient data, including genomics and proteomics, to identify specific treatment options tailored to the patient's unique characteristics. This potential for personalized treatments presents a significant opportunity for improved patient outcomes and targeted therapies.
- Improving Clinical Trials: AI can improve the design and efficiency of clinical trials by optimizing patient recruitment, selecting suitable trial endpoints, and identifying potential safety issues. By enhancing the clinical trial process, AI can help reduce costs and accelerate the development of new treatments.
- Enhancing Disease Diagnosis and Management: AI-powered tools can improve disease diagnosis by analyzing medical imaging data, genomic information, and clinical data. AI algorithms can detect patterns and anomalies that may not be easily identified by human experts, leading to earlier and more accurate diagnoses.
- Automation and Efficiency: AI can automate repetitive and time-consuming tasks in biotech research, such as data analysis, image processing, and lab workflows. This allows researchers to focus on higher-level tasks and accelerates the pace of scientific discovery.
- Partnerships and Collaborations: Investors are interested in AI-powered biotech companies that actively collaborate with academic institutions, pharmaceutical companies, and other industry players. These partnerships enhance the potential for cross-pollination of expertise, data sharing, and accelerated development of innovative solutions.
- Market Potential and Competitive Advantage: Investors recognize the market potential of AI-powered biotech solutions and the competitive advantage they can bring to companies in the sector. The ability to leverage AI technologies effectively can lead to breakthrough discoveries, improved patient outcomes, and a strong market position.
Biotech has shown immense potential in 2023 to revolutionize drug discovery, enable personalized medicine, improve clinical trials, enhance disease diagnosis and management, drive automation and efficiency, foster collaborations, and create market opportunities. The combination of AI and biotech holds great promise for transforming healthcare and delivering innovative solutions to address unmet medical needs.
Impact of AI on these domains of biotech: neurodegenerative diseases, immuno-oncology, cardiovascular diseases, metabolic diseases?
- Neurodegenerative Diseases: AI has the potential to significantly impact the diagnosis, treatment, and management of neurodegenerative diseases such as Alzheimer's, Parkinson's, and Huntington's disease. AI algorithms can analyze large datasets of patient information, imaging data, and genetic data to identify disease biomarkers, improve early detection, and aid in the development of targeted therapies.
- Immuno-oncology: AI is being used in immuno-oncology to analyze complex interactions between the immune system and cancer cells. It can help identify new immunotherapy targets, predict patient response to treatment, and optimize treatment regimens. AI can also assist in the discovery of novel combination therapies to enhance the effectiveness of immunotherapies.
- Cardiovascular Diseases: AI has the potential to impact cardiovascular disease research and treatment by analyzing large-scale patient data, including electronic health records, medical imaging, and genomic data. AI algorithms can assist in early detection of cardiovascular diseases, predict disease progression, guide treatment decisions, and optimize personalized therapies.
- Metabolic Diseases: AI can contribute to the understanding and management of metabolic diseases such as diabetes, obesity, and metabolic syndrome. AI algorithms can analyze diverse data sources, including patient health records, wearable devices, and genomic data, to develop personalized interventions, predict disease progression, and optimize treatment strategies
What is the CAGR for the AI in Drug Discovery Market in Japan and South Korea?
Research report by MarketsandMarkets, the AI in Drug Discovery Market in Japan and South Korea is projected to grow at a CAGR of 45.7% from 2022 to 2027. AI-powered drug discovery platforms are being utilized to identify new drug targets, design drugs, and optimize the drug development process. These platforms can screen large datasets of molecules and predict the efficacy and toxicity of drugs. The market growth is driven by rising investments in healthcare, the increasing prevalence of chronic diseases, and government support for technology development in the healthcare sector. The market can be segmented by technology, application, end-user, and indication. Machine learning is expected to be the largest segment, while target identification is anticipated to be the fastest-growing segment. Key players in the market include Almac Discovery, Insilico Medicine, and Exscientia. These companies have shown success in developing drugs using AI-powered platforms. The market presents opportunities for businesses in the AI in Drug Discovery space to leverage crucial industry insights and benefit from the growing demand.
What is the CAGR for the AI in Drug Discovery Market in the USA?
The projected CAGR for the AI in Drug Discovery Market in the USA, according to the research report by MarketsandMarkets, is 45.7% from 2022 to 2027. This indicates significant growth opportunities in the market. Rising investments in healthcare, the increasing prevalence of chronic diseases, and government support are key factors driving this growth.
The USA is the world's largest healthcare spender, with substantial investments being made in the development of new drugs. The rising prevalence of chronic diseases such as cancer, diabetes, and cardiovascular diseases further contributes to the demand for innovative drugs. The US Food and Drug Administration (FDA) has also been supportive of the development of new technologies in healthcare, including AI-powered drug discovery.
In the USA, companies such as Insilico Medicine, Exscientia, and Recursion Pharmaceuticals are playing a prominent role in the AI in Drug Discovery Market. These companies utilize AI to design new drugs, screen large datasets of molecules, predict drug efficacy and toxicity, and identify new drug targets.
The combination of factors such as increased investments, the burden of chronic diseases, and regulatory support provides a favorable environment for the growth of the AI in Drug Discovery Market in the USA. It presents valuable business opportunities for companies operating in this space to leverage the forecasted growth and contribute to advancements in drug discovery.
How does generative AI in biology contribute to the discovery and design of novel molecules and compounds?
Generative AI in biology, particularly in the field of drug discovery, is contributing to the discovery and design of novel molecules and compounds in several ways:
- Compound Discovery and Design: AI is being increasingly used in medicinal chemistry, supported by computer-aided drug design and cheminformatics. Computational methods have long been employed to aid in the search for and optimization of active compounds. AI has shown promise to impact medicinal chemistry in areas such as compound design and synthesis. Machine learning (ML) and deep learning (DL) methods, such as deep neural network (DNN) architectures and learning strategies, are being used for molecular property prediction and virtual compound screening.
- Synthetic Chemistry: AI is being used in computer-aided synthesis planning (CASP) to accelerate the decision-making process by which medicinal chemists choose the most appropriate routes to synthesize new compounds. AI can predict chemical reactions and their success rates, which is highly relevant for drug discovery. This includes both retrosynthetic and forward reaction prediction.
- Compound Design: Generative de novo design via DNNs aims at proposing structurally novel molecules with desired properties such as potency, suitable drug metabolism and pharmacokinetics (DMPK) profiles, or synthetic accessibility. Generative models are typically trained on SMILES notations to infer chemical syntax for compound generation.
- Uncertainty Estimation and Active Learning: Uncertainty estimation for ML predictions is another important area of research that has the potential to significantly impact ML in medicinal chemistry. Different frameworks for uncertainty estimation have been reported, such as frequentist analysis or Bayesian approaches.
- Integration with Automated Synthesis: AI is being integrated with fully automated synthesis capacity. For example, one study combined generative design with a microfluidics platform for on-chip chemical synthesis. DNN model fine-tuning yielded novel bioactive candidates for single-step reactions via the microfluidics-assisted synthesis platform.
AI is playing a crucial role in accelerating drug discovery by aiding in the design and synthesis of novel molecules and compounds, predicting chemical reactions, estimating uncertainty in predictions, and integrating with automated synthesis platforms. However, it's important to note that while AI provides valuable tools and insights, the ultimate decisions about new or further-improved active compounds are made by investigators with the aid of ML/DL outputs.
What are the applications of generative AI in drug discovery and development processes in biotechnology?
Generative AI has several applications in the drug discovery and development processes in biotechnology:
- Drug Discovery: AI can help in the discovery of new drugs by analyzing large datasets of chemical compounds and their effects. It can predict the properties of potential drugs and identify promising candidates for further testing. AI can also generate new molecular structures that could potentially act as drugs.
- Drug Development: Once a potential drug has been identified, AI can assist in its development. This includes optimizing the drug's structure to improve its effectiveness, reduce side effects, and make it easier to produce. AI can also predict how the drug will interact with various biological systems, helping to anticipate and mitigate potential issues.
- Personalized Medicine: AI can help tailor treatments to individual patients based on their unique genetic makeup and health status. This can improve the effectiveness of treatments and reduce side effects.
- Clinical Trials: AI can assist in the design and analysis of clinical trials, helping to determine the most effective doses and identify potential side effects. It can also help to identify suitable participants for trials.
- Manufacturing: AI can help optimize the manufacturing process for drugs, improving efficiency and reducing costs. It can also help ensure the quality and consistency of drugs.
- Post-Market Surveillance: After a drug has been approved and is on the market, AI can help monitor its use and identify any unexpected side effects or issues.
AI is becoming an essential tool in all stages of the drug discovery and development process, from the initial identification of potential drugs to their production and post-market surveillance.
How is generative AI utilized in the field of synthetic biology to design and engineer new biological systems?
Generative AI is utilized in the field of synthetic biology in several ways:
- Predictive Models and Simulations: AI is used to develop predictive models and simulations, which can be used to design and optimize biological systems. Machine learning algorithms can be used to analyze large amounts of data and predict the behavior of biological systems.
- Automated Laboratory Techniques: AI is used to develop automated laboratory techniques, which can be used to perform complex procedures and experiments. Robotics and automation are often used in synthetic biology to perform repetitive and time-consuming tasks such as DNA assembly, gene editing, and protein expression.
- Protein Structure Prediction: One of the most notable examples of AI in synthetic biology is AlphaFold, a deep-learning model developed by DeepMind. AlphaFold can predict the 3D structure of proteins from their amino acid sequence, which is an essential task in the field of structural biology. The ability to predict protein structure is crucial in understanding how proteins work and using this information for drug development, protein engineering, and many other biotechnology applications.
- Integration with Other Fields: The field of synthetic biology is emerging as a powerful tool for the study of biological systems. It is also a growing field that integrates with other fields to create new technologies. Some of these disciplines include data handling, which is critical to many scientific and technological fields, including synthetic biology and essential for developing new technologies.
Synthetic biology is a rapidly growing field of technology that holds tremendous potential for future commercial and industrial applications. The promise of this technology can be summarized by the ability for rapid product prototyping, systems-level analysis, minimal impact on the environment, and reduced costs associated with goods production. However, it also brings concerns and challenges in terms of ethical and societal implications, which will need to be considered.
In terms of regulation, synthetic biology is regulated by both agencies at the federal and state level through the US Food and Drug Administration (FDA) and Environmental Protection Agency (EPA). European Union member states have also taken steps to regulate synthetic biology products under existing EU regulations governing genetically modified organisms (GMOs). There are also several international efforts to regulate synthetic biology and ensure that it is developed and used in a safe and responsible manner.
Scientists involved in the research and development of synthetic biology are responsible for driving new innovations in the field and ensuring that the technology is used responsibly and ethically. Both the scientific community and governments recognize the importance of self-regulation in the field of synthetic biology, and scientists have a responsibility to conduct their research with integrity and in accordance with guidelines established by professional organizations and institutions.
Overall, the future of synthetic biology depends on asking questions about its potential risks and benefits, ensuring responsible development, preparing for future challenges—and sparking new ideas. Synthetic biology should be developed in a way that respects society's values and interests and the concerns of its citizens about how new technologies will affect their lives. By asking questions about the future of synthetic biology, we can ensure that it is developed and used in a responsible and ethical manner and find solutions to the challenges posed by this new technology.
In what ways does generative AI support protein folding prediction and structure determination in biotechnology research?
Generative AI, particularly deep learning models, have shown significant promise in supporting protein folding prediction and structure determination in biotechnology research. Here are some ways in which it is being utilized:
- Protein Structure Prediction: Generative AI models can infer the three-dimensional structure of a protein from its amino acid sequence. This is crucial as the structure of a protein is vital for understanding its function. The process involves predicting the secondary and tertiary structure from the primary structure of the protein.
- AlphaFold: An example of a deep learning model used in protein structure prediction is AlphaFold, developed by DeepMind Technologies. AlphaFold predicts the 3D structure of proteins, which are essential in the functioning of cells. The model was trained on known models of proteins and learned to predict proteins on its own. This has greatly accelerated the effort to model proteins, a problem that has been under pursuit for decades.
- Database of Predicted Protein Structures: DeepMind has released an AlphaFold database with predicted structures for a large number of proteins, including all those in the human body. This allows researchers and labs around the world to make use of it for various purposes. The database has been expanded to include nearly all the proteins known to science, including proteins found in animals, plants, bacteria, and many other organisms.
- Applications in Disease Research: The AlphaFold model has been used in research into diseases such as malaria. For instance, scientists at the University of Oxford used AlphaFold to advance their research into vaccines to stop the spread of malaria. They used the model to get a detailed view of a malaria protein, which was not possible with older methods.
Generative AI is playing a crucial role in protein folding prediction and structure determination, which are key areas in biotechnology research. The ability to predict protein structures can lead to significant advancements in understanding biological functions and in the development of new drugs and treatments.
What future developments and trends can be anticipated in the application of generative AI in biology and biotechnology?
- Accelerated Drug Discovery: Generative AI is expected to play a significant role in accelerating the drug discovery process. AI algorithms can generate novel molecules and predict their properties, enabling researchers to identify potential drug candidates more efficiently. This can lead to the discovery of new therapies for various diseases.
- Customized Drug Design: Generative AI has the potential to revolutionize personalized medicine by enabling the design of drugs tailored to individual patients. AI algorithms can analyze genomic and proteomic data to identify specific molecular targets and design drugs that interact with them, offering more precise and effective treatments.
- Design of Enzymes and Proteins: Generative AI can aid in the design of enzymes and proteins with specific functions. By utilizing deep learning algorithms, AI models can generate protein sequences and predict their structural properties, leading to the creation of bioengineered proteins with desired characteristics for various applications, such as industrial processes or therapeutic interventions.
- Bioengineering and Synthetic Biology: Generative AI can facilitate the design and engineering of new biological systems in synthetic biology. AI algorithms can assist in the optimization of genetic circuits, the prediction of metabolic pathways, and the creation of novel genetic constructs. This can accelerate the development of bio-based products, biomanufacturing processes, and bioengineered organisms.
- Biomedical Image Analysis: Generative AI techniques, such as deep learning, are increasingly being applied to analyze biomedical images. AI models can assist in image segmentation, disease detection, and image-based diagnostics. This can enhance medical imaging technologies and support clinicians in making accurate diagnoses.
- Data Integration and Knowledge Discovery: Generative AI can help integrate and analyze vast amounts of biological data from diverse sources. AI algorithms can uncover hidden patterns, relationships, and insights from complex biological datasets, facilitating knowledge discovery and advancing our understanding of biological processes.
These are some of the anticipated future developments and trends in the application of generative AI in biology and biotechnology.
GET AHEAD
Top Research Reports to Fuel Your Industry Knowledge- Artificial Intelligence (AI) Market by Offering (Hardware, Software), Technology (ML (Deep Learning (LLM, Transformers (GPT 1, 2, 3, 4)), NLP, Computer Vision), Business Function, Vertical, and Region - Global Forecast to 2030
- AI in Pathology Market by Component (Software, Scanners), Neural Network (CNN, GAN, RNN), Application (Drug Discovery, Diagnosis, Prognosis, Workflow, Education), End User (Pharma, Biotech, Hospital Labs, Research), & Region - Global Forecast to 2028
- Artificial Intelligence / AI in Drug Discovery Market Size by Offering, Process (Target selection, Validation, Lead generation, optimization), Drug Design (Small molecule, Vaccine, Antibody, PK/PD), Dry Lab, Wet Lab (Single Cell analysis) & Region - Global Forecast to 2028
Editor's Pick
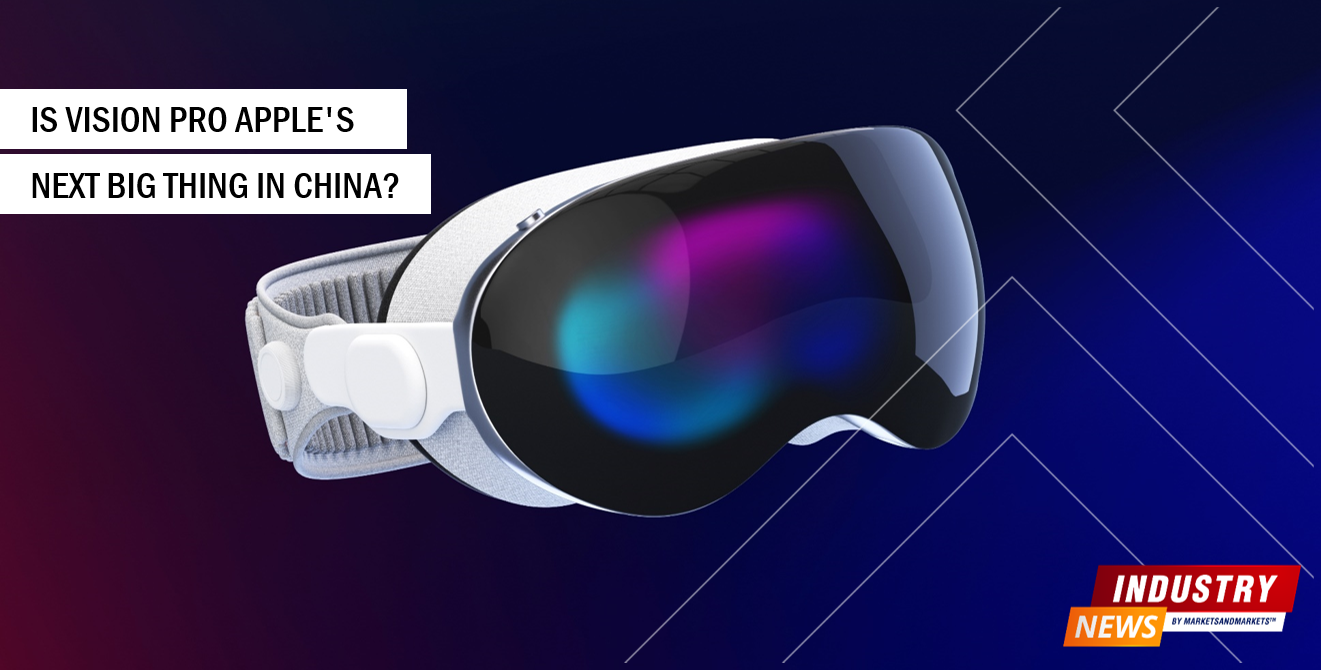
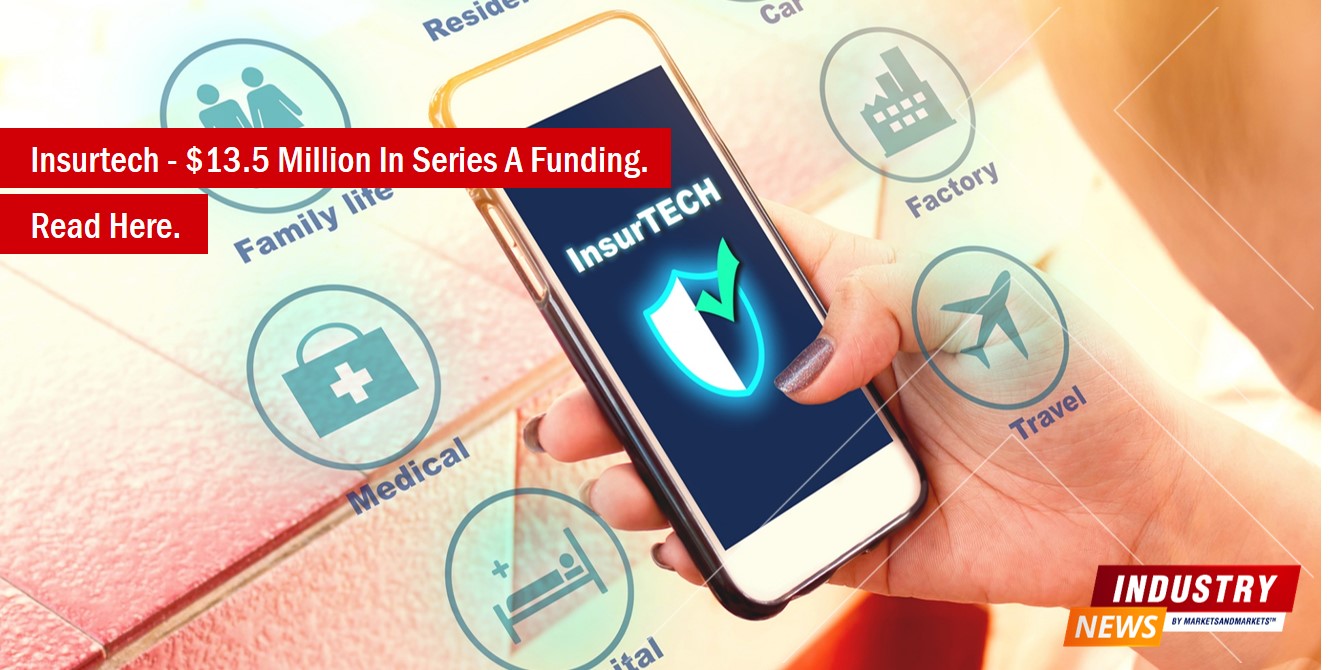
Information and Communication Technology
Insurtech Funding News - Coverdash raises USD 13.5 Million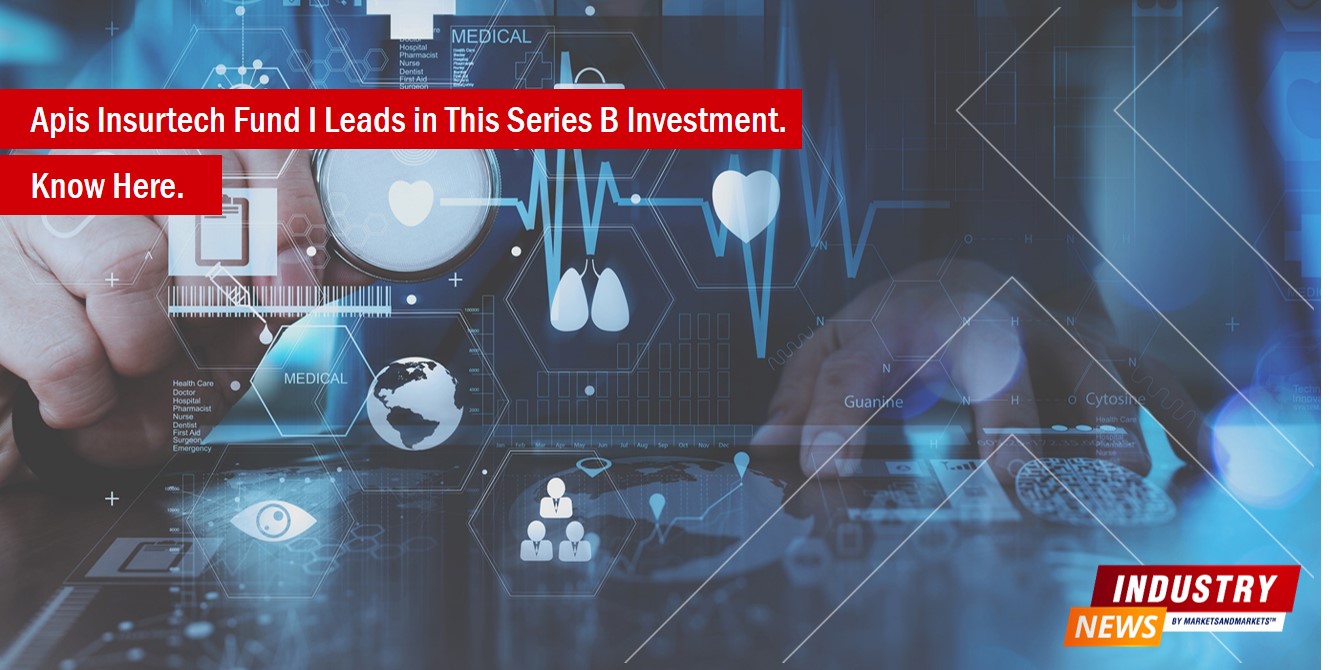
PODCASTS
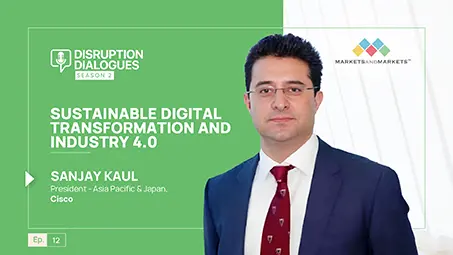
Sustainable Digital Transformation & Industry 4.0
Sanjay Kaul, President-Asia Pacific & Japan, Cisco, and host Aashish Mehra, Chief Research Officer, MarketsandMarkets, in conversation on unraveling 'Sustainable Digital Transformation and Industry 4.0'
11 July 2023|S2E12|Listen Now
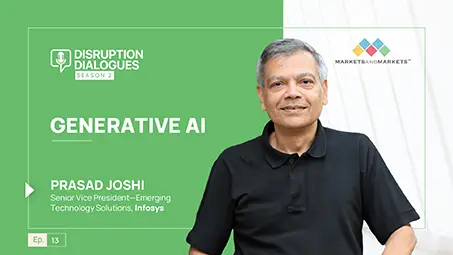
Generative AI
Prasad Joshi, Senior Vice President-Emerging Technology Solutions, Infosys, and host, Vinod Chikkareddy, CCO, MarketsandMarkets, in exploring the recent advances in AI and the generative AI space.
7 Nov 2023|S2E13|Listen Now
AI in Drug Discovery Market


Download Whitepaper
Investors are keenly interested in AI powered Biotech
The combination of AI and biotech holds great promise for transforming healthcare and delivering innovative solutions to address unmet medical needs.
In the USA, companies such as Insilico Medicine, Exscientia, and Recursion Pharmaceuticals are playing a prominent role in the AI in Drug Discovery Market.
