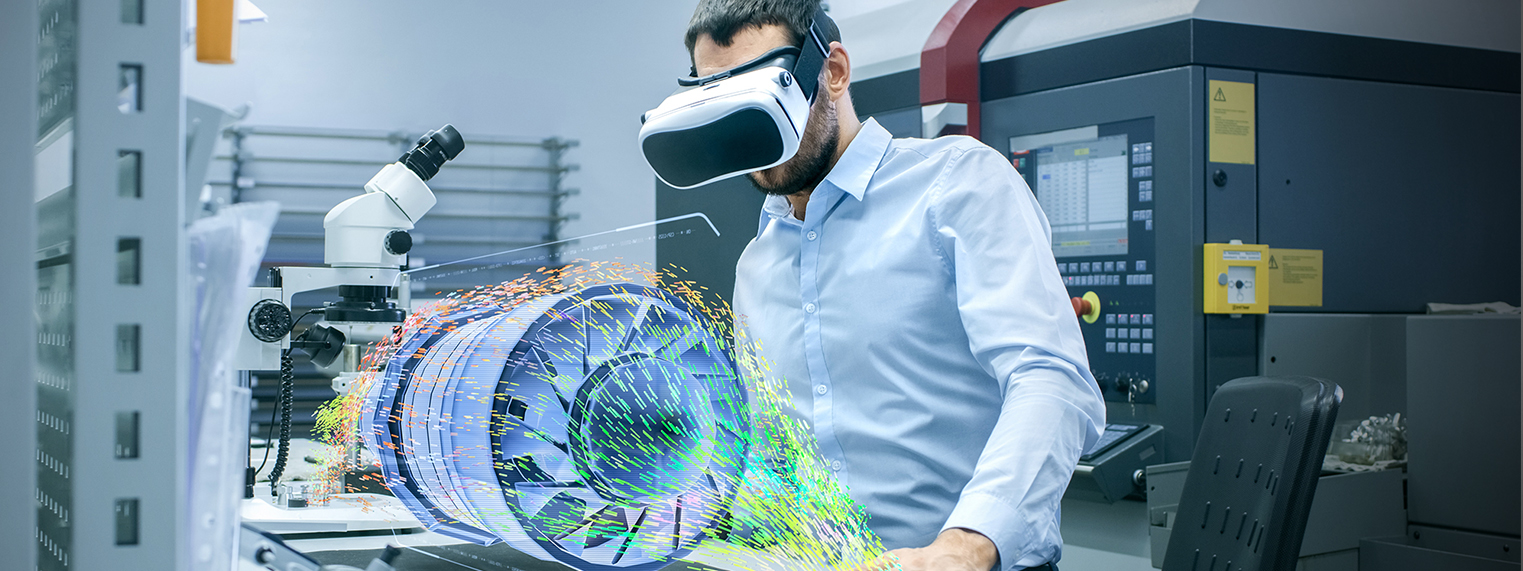
Digital Twin Revolution: Unveiling the Virtual Mirror of Reality Across Industries
What is Digital Twin
A digital twin is a virtual replica of a physical entity, utilizing embedded sensors to gather real-time data. This data, encompassing factors such as energy output, temperature, and weather conditions, is transmitted to a processing system, thereby refining the accuracy of the digital twin. The virtual model can be utilized for running simulations, examining performance issues, and devising enhancements, offering valuable insights that can be applied to the actual physical object.
Access our popular detailed report here:
https://www.marketsandmarkets.com/Market-Reports/digital-twin-market-225269522.html
Digital twins go beyond individual objects, capturing entire lifecycles using real-time sensor data for simulation and monitoring. This versatile technology reproduces various items, from individual factory equipment to expansive installations such as wind turbines or entire cities. By monitoring asset performance, detecting faults, and providing insights for maintenance decisions, digital twins play a crucial role in improving efficiency and facilitating informed decision-making throughout the lifecycle of an asset.
The idea of a digital twin covers a broad spectrum, encompassing various representations of physical objects, processes, services, or environments. These virtual counterparts can span from jet engines and wind farms to complete cities. Operating as computer programs, digital twins utilize real-world data to simulate and forecast the performance of a product or process. Their integration with the Internet of Things (IoT), artificial intelligence, and software analytics amplifies their capabilities, making them crucial in contemporary engineering, fostering innovation, and averting costly failures.
Download PDF Brochure @
https://www.marketsandmarkets.com/pdfdownloadNew.asp?id=225269522
Digital twins gather and integrate diverse data types, such as physical, manufacturing, and operational data. A virtual mirror model is generated through AI algorithms and analytics software, maintaining synchronization with the physical asset. The fundamental architecture includes a model that mirrors the real-world system, IoT sensors for real-time data transmission, data synchronization mechanisms, and data monitoring and analysis software. Digital twins offer nearly precise operational representations of assets by combining modeling and analytics for specific objectives.
The process of developing a digital twin includes capturing data from physical assets through sensors, transforming it into a digital record, employing advanced analytics and AI for extracting meaningful insights, and utilizing algorithms to translate digital decisions into impactful actions in the physical realm.
Digital Twin Ecosystem
Source: Marketsandmarkets
Importance of digital twin
Digital twins are virtual replicas of physical objects, processes, or systems, playing a pivotal role across various industries. The fundamental advantage of digital twins is their contribution to informed decision-making. Organizations can engage in simulations and predictive analyses by creating a virtual model mirroring the physical object or system, leading to more profound insights and efficient decision-making. This proves particularly valuable in product development, as digital twins empower companies to test and validate new concepts and designs within a virtual environment before committing resources to physical prototypes.
Moreover, digital twins play a crucial role in streamlining maintenance and operations. Through the continuous collection and analysis of real-time data from the physical asset, organizations can detect potential issues, predict maintenance requirements, and enhance overall performance. This proactive approach has the potential to result in cost savings, heightened efficiency, and minimized downtime.
So, the significance of digital twins is evident in their capacity to refine decision-making, expedite product development, and optimize maintenance and operations. As technology progresses, its influence is anticipated to expand across diverse industries, solidifying its status as an increasingly valuable asset for organizations seeking to enhance their processes and offerings.
How digital twin is different from simulation
Digital twins and simulations are based on virtual models, yet they fulfill different objectives and exhibit distinct characteristics. Here are the primary distinctions between the two:
Difference Between Digital Twin and Simulation
Particulars |
Digital twins |
Simulation |
Purpose |
Digital twins are digital representations of physical objects, processes, or services. |
Simulations are used to study specific processes or systems. |
Data |
Digital twins utilize real-time data to update the virtual model continually. |
Simulations generally rely on predetermined data sets and may not integrate real-time data. |
Cross-functional integration |
Digital twins are crafted to streamline the entire lifecycle of equipment, products, and other entities more efficiently. |
Simulations might lack an equivalent level of cross-functional integration. |
Scope |
Digital twins can execute numerous valuable simulations to analyze various processes. |
Simulations usually study one particular process. |
Real-Time Monitoring |
Digital twins offer straightforward monitoring of intricate systems containing hundreds or thousands of assets. |
Simulations might not possess comparable real-time monitoring capabilities. |
Predictive maintenance |
Leveraging digital twins can enhance operations by predicting maintenance needs, minimizing downtimes, and improving employee safety. |
Simulations might lack equivalent predictive capabilities. |
Note: The information provided above represents and does not encompass the entire ecosystem of Digital Twins.
Digital twins offer greater comprehensiveness and versatility by supplying real-time data and facilitating cross-functional integration. In contrast, simulations tend to concentrate on analyzing specific processes and may lack real-time data integration. The selection between a digital twin and a simulation hinges on the particular requirements and goals of the project.
80% of the Forbes Global 2000 B2B companies rely on MarketsandMarkets to identify growth opportunities in emerging technologies and use cases that will have a positive revenue impact.
- Leading Automated Guided Vehicle Companies 2024: An In-depth Analysis
- CHARGED UP: SHIFT TO E-MOBILITY AND THE EVOLUTION OF TRANSPORTATION
- Global Automotive Market: Predictions For 2024
- Revolutionizing Depot Charging: Hockey Stick Growth on the Cards
- The Future of Silicon Battery Industry: Innovations and Market Outlook
Brief historical background of Digital Twin
The digital twin concept traces its roots back to the 1960s, gaining formal acknowledgment in 2002 during a presentation at the University of Michigan by Michael Grieves. This presentation detailed the establishment of a product lifecycle management center, emphasizing the integration of real and virtual spaces and the exchange of data between the two realms. Although the terminology has evolved over the years, the fundamental concept of creating a unified entity comprising both a digital and physical twin has remained consistent since its inception. NASA had been employing basic twinning concepts in space programming since the 1960s, exemplified by the replication of physical systems on Earth to mirror those in space, as illustrated in the case of Apollo 13.
In 1970, the Apollo 13 mission encountered a crisis when oxygen tanks exploded early in the mission. Despite being 200,000 miles away, NASA's digital twin model of Apollo 13 on Earth was pivotal in the rescue mission. This early utilization of digital twin technology empowered engineers to test solutions from the ground level and address issues remotely. Over time, the evolution of digital twins shifted from physical to predominantly virtual simulations. The term "digital twin" was initially introduced in 1998, referring to a digital replica of actor Alan Alda's voice. It was only in 2017 that digital twin technology emerged as one of the top strategic technology trends, owing to the cost-effectiveness facilitated by the Internet of Things.
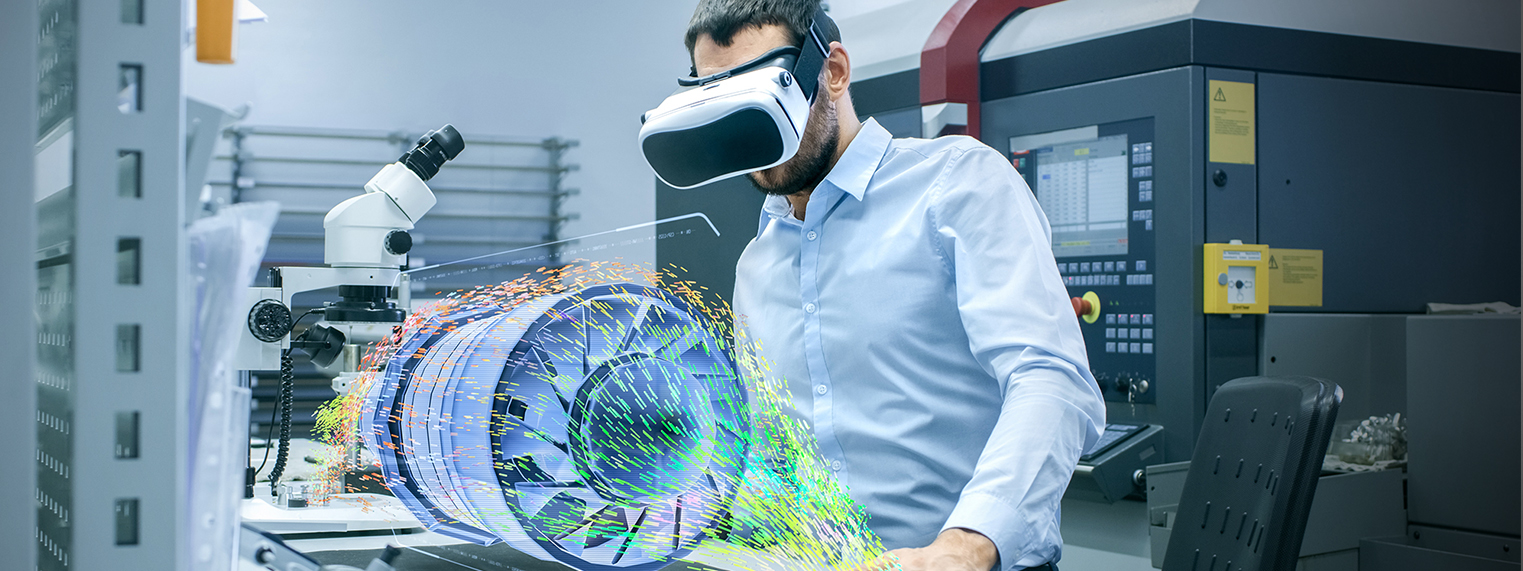
What are the driving and challenging factors of the market?
The market dynamics are shaped by both driving forces and challenges. One significant driver is the revolutionary impact of digital twins on manufacturing, offering a strategic approach for proactive defect detection and efficient supply chain management. Meanwhile, the widespread adoption of digital twins for predictive maintenance stands out as another driver, optimizing maintenance processes and predicting failures for enhanced operational efficiency. However, challenges such as data fragmentation, quality concerns, and integration complexities hinder the seamless implementation of digital twins.
Revolutionizing Manufacturing with Digital Twins: A Strategic Approach to Proactive Defect Detection and Efficient Supply Chain Management
Manufacturers grapple with substantial challenges from unplanned downtime and production waste, compelling them to seek a system capable of proactively identifying potential defects and failures to mitigate future losses. Adopting digital twin technology offers a solution to reduce the excessive time and costs associated with manufacturing. By implementing digital twins, engineers can enhance a product's performance by continuously modifying the physical prototype throughout the design phase. As a result, a digital prototype, created in tandem with a digital twin, facilitates efficient simulations and modifications at any stage, significantly minimizing both time and costs. Digital twins find extensive applications in manufacturing, encompassing configuration management, asset management, process control, performance management, and simulation modeling.
Digital twin technology primarily depends on historical data, sometimes incorporating real-time data. Analysts utilize digital twins to deepen their understanding of supply chain behavior, foresee unusual circumstances, and develop action plans to reduce costs and enhance process efficiency. By applying digital twins, companies can pinpoint trends and simulate the impacts of changes in various processes, thereby refining supply chain design and testing. Furthermore, they can monitor risks and assess probabilities for well-informed decision-making. Digital twins offer a seamless, end-to-end perspective of all processes and bottlenecks within the supply chain, allowing manufacturers to address issues with minimal human intervention swiftly. These digital twins gather data to reveal potential flaws at every delivery stage. River Logic, a U.S.-based company specializing in prescriptive analytics and optimization, provides its customers with a supply chain digital twin, addressing decision-making challenges across short, medium, and long-term scenarios. Similarly, SAP SE (Germany), in collaboration with Siemens (Germany), jointly markets a digital twin focused on production and supply chains.
Optimizing Maintenance Processes Through Widespread Adoption of Digital Twins for Predictive Maintenance
?The extensive adoption of digital twins for predictive maintenance is evident across diverse industries. Predictive maintenance, anchored in digital twins, entails gathering real-time sensor data to assess the condition and performance of a product, process, or system. This data is then analyzed alongside historical records of failure modes and their criticality. The insights gleaned from this analysis are applied to anticipate maintenance needs. Going beyond predicting failures, this approach provides several advantages that enhance the overall maintenance process. Digital twins enable the computation of key performance indicators related to maintenance and can forecast the performance of a system under varying conditions. Acting as accurate real-time models of a product, digital twins facilitate simulations and predictions concerning specific factors like runtime, exposure to extreme operating conditions, and temperature.
By integrating with augmented and virtual reality, digital twins enhance engagement in maintenance, reducing reliance on costly adjustments in later stages and resulting in time and cost savings. In the aerospace industry, digital twins are crucial in minimizing maintenance expenses, optimizing operations, and decreasing downtime. General Electric (GE) has specifically developed digital twins for aircraft landing gears in the United States. This involves the placement of sensors at critical failure points on the landing gear, enabling the prediction of malfunctions and the diagnosis of the landing gear's lifecycle. Additionally, GE Aviation has developed a digital twin for every engine it produces. This initiative provides the engine manufacturer with a digital simulation for each engine, enabling GE to anticipate potential part failures.
Challenges impacting the development of Digital Twin
Digital twins, virtual counterparts of physical assets, hold the potential to revolutionize industries spanning manufacturing to healthcare. Despite their considerable promise, several obstacles impede their widespread adoption. Two critical challenges hinder the Digital Twin revolution:
Data: The cornerstone of any Digital Twin is data. Real-time, precise data from sensors, simulations, and historical records is essential to construct a faithful digital replica. Yet, achieving this ideal data ecosystem remains challenging:
Data Fragmentation: Information may be isolated across different departments, systems, and formats, complicating the gathering, integration, and harmonization process. This inconsistency impedes the creation of a unified depiction of the asset.
Data Quality Concerns: Even when data is accessible, issues such as accuracy, noise, and missing values can compromise its quality. Using unreliable data in the Digital Twin results in flawed simulations and inaccurate predictions.
Real-time Challenges: Capturing and transmitting real-time data streams proves complex and costly, particularly for large or geographically dispersed assets. Limited bandwidth and latency issues further hinder the development of truly dynamic Digital Twins.
Addressing these data challenges necessitates robust data management strategies, standardization efforts, and investments in reliable sensor infrastructure.
Integration: Digital Twins exist within a broader software, hardware, and human user ecosystem. Seamless integration with these elements is crucial for unlocking their full potential:
Legacy Systems: Many industries rely on legacy systems with limited connectivity and data-sharing capabilities. Integrating Digital Twins with these systems can be cumbersome and costly.
Interoperability: The absence of standardized data formats and communication protocols across different platforms creates interoperability hurdles, making it challenging to share and exchange data between Digital Twins and limiting their collaborative value.
Skills Gap: Effectively implementing and utilizing Digital Twins demands a workforce with specialized data analysis, AI, and digital modeling skills. Bridging this skills gap requires training programs and talent development initiatives.
Tackling these integration challenges necessitates open-source solutions, standardized protocols, and investments in upskilling the workforce. While these challenges are formidable, ongoing research and development efforts pave the way for solutions. Continued advancements in data management, interoperability, and skills development suggest that Digital Twins are on the cusp of overcoming these hurdles, ushering in a new era of industrial efficiency and innovation.
Integration of Digital Twin and Artificial Intelligence (AI)
The implementation of AI in Digital Twins is a transformative force across industries. From manufacturing to sustainability practices, AI enhances predictive maintenance, optimizes processes, and contributes to the broader goals of efficiency and sustainability. The synergy between AI and Digital Twins continues to evolve, promising a future where virtual replicas and real-world applications seamlessly integrate for unprecedented advancements.
AI-Driven Digital Twins in Healthcare: A Companion for a Lifetime
The impact of AI on healthcare transcends traditional boundaries, introducing a fascinating concept - the AI-driven Digital Twin. This innovative approach transforms AI into a digital companion for individuals and their loved ones, including doctors. This groundbreaking idea opens avenues for engaging with AI-generated versions based on unique imperfections and behavior patterns, challenging the limits of companionship and guidance in the digital age.
Imagine interacting with a digital twin of a loved one, whether a doctor or a mother. This AI-powered twin responds based on real data, capturing the essence of their persona. The potential of this digital twin companion extends beyond personal interactions, reaching into professional and educational realms. In healthcare, a doctor's digital twin could be a virtual advisor, assisting decision-making and providing personalized treatment recommendations.
However, the incorporation of AI-driven digital twins in healthcare raises ethical considerations. Privacy, consent, and the potential for emotional manipulation must be navigated with care. Creating digital replicas without explicit consent infringes upon privacy rights, and replicating the voice and behaviors of a deceased loved one raises emotional concerns.
AI-Powered Digital Twins: Revolutionizing Modern Manufacturing
In the rapidly evolving landscape of modern manufacturing, the integration of AI-driven digital twins stands out as a transformative force. This merging of digital model simulation and manufacturing AI results in virtual replicas of physical assets, providing real-time monitoring, analysis, and optimization. The potential impact is substantial, addressing the critical need for efficiency as underscored by significant downtime losses reported by manufacturers.
A foundational understanding of digital twins is essential before exploring AI integration. These digital replicas, encompassing individual machine parts to entire ecosystems, continuously receive real-time data from their physical counterparts. AI elevates digital twins from passive reflectors to proactive learning systems capable of predictive analysis and real-time decision-making.
AI enriches digital twins by processing extensive data from diverse sources, forecasting maintenance needs, and facilitating real-time insights. The capacity to process and analyze data in real-time enables digital twins to represent current states and predict future conditions accurately. AI-driven digital twins enhance accuracy and functionality through continuous learning from processed data.
Conclusion: Navigating the Future with AI-Driven Digital Twins
Integrating AI with Digital Twins represents a transformative influence, restructuring industries, streamlining processes, and redefining interactions between humans and machines. The potential applications span various sectors, such as healthcare and manufacturing. However, ethical considerations should govern the implementation, ensuring transparency, consent, and the preservation of individual rights.
As we venture into the future, the dynamic synergy between AI and digital twins promises unprecedented advancements. Through ongoing research, continuous learning, and a commitment to responsible deployment, AI-driven digital twins can propel us into a future where processes are optimized, resilient, and adaptive. This trajectory sets new industry standards characterized by precision and efficiency.
Application of Digital Twin
Automotive
In the automotive sector, digital twins boost productivity by generating virtual replicas of physical entities. Manufacturers utilize these virtual counterparts to evaluate performance in various scenarios, detect flaws, and conduct simulations, ultimately reducing product failures and refining the development process. Real-time monitoring through digital twins enhances production efficiency by managing products and systems utilizing feedback data from sensors for predictive maintenance. Moreover, digital twins serve educational purposes, enabling remote training without a physical setup. Major automotive manufacturers like Renault, Ford, and Tesla have adopted digital twin technology to optimize their processes. Renault employs virtual models in the design phase, conducting tests and incorporating real-world usage data to streamline their workflow and reduce the time required for designing new vehicles. Ford utilizes digital twin technology for product design enhancements, exemplified by developing a headlight system that alerts drivers when approaching corners. Tesla integrates digital twin technology by creating a virtual replica for each car it produces, enabling continuous performance monitoring and timely identification of maintenance needs. These instances underscore the significant role of digital twin technology in enhancing efficiency, product design, and maintenance within the automotive manufacturing sector.
Energy and Utility
Digital Twin can be implemented for various end-users of energy and utility. Below, we have discussed briefly solar and wind farms.
Wind farm companies that use digital twin technology, including Doosan Heavy Industries & Construction, partnered with Microsoft and Bentley Systems to develop a digital twin of their wind farms.
The use of Digital Twins in the solar farm sector has proven revolutionary. Operating as a virtual replica of an actual solar power plant, a Digital Twin facilitates experimentation, hypothesis testing, and predictive behavior analysis. By adjusting input variables, Digital Twins play a crucial role in averting equipment failures, optimizing system design, and reducing operating costs. Solar Asset Managers benefit significantly from Digital Twins, enabling them to swiftly compare a PV plant's current performance with its expected performance derived from its digital counterpart, utilizing real-time plant data, irradiance, and temperature. This advanced technology enhances real-time performance and loss analysis, streamlining daily tasks, boosting solar plant uptime, and efficiently prioritizing maintenance activities.
Construction
Digital twins are revolutionizing the construction industry by offering innovative solutions to real-world challenges. By creating virtual replicas of physical spaces during the early planning and design stages, digital twins enable efficient collaboration and communication among stakeholders. These 3D models, combining data such as 3D models, sensor data, and real-time performance data, help simulate and optimize material choices, energy use, and maintenance schedules. The construction industry benefits from increased efficiency, improved safety, enhanced accuracy, and quality assurance throughout the construction lifecycle. Despite the transformative potential, challenges such as data overload, integration hurdles, and high initial costs need to be addressed, emphasizing the importance of implementing best practices to optimize the use of digital twin technology. Companies such as SrinSoft, Ansys, and Matterport are actively involved in developing Digital Twin for the construction industry.
Oil and Gas
Digital twins benefit the oil and gas sectors by serving as a secure repository for asset documentation, addressing workforce aging concerns, and boosting operational efficiency. Through the early detection of equipment failure, simulation of drilling scenarios, and real-time data collection from sensors, digital twins enable proactive maintenance, reduce downtime, and diminish the need for specialized personnel. The successful implementation of digital twins, driven by AI, ML, and IoT, hinges on a robust cellular network, particularly leveraging 4G and 5G technologies, to manage extensive data transfer with minimal latency. Companies like Cognite and Kongsberg Digital actively contribute to developing digital twins in the Oil and Gas ecosystem, receiving funding from Aramco and Shell, respectively.
Healthcare
Digital twins, gaining rapid traction across diverse industries, significantly influence the healthcare sector. These virtual replicas, encompassing entities from patients to hospitals, utilize electronic health records, disease registries, and various omics data to present a comprehensive perspective on health-related entities and processes. Fueled by IoT and AI, healthcare digital twins enable real-time data connectivity, providing insights derived from behavioral, biometric, cognitive, and psychological information.
The benefits of employing digital twins in healthcare are extensive: improving patient care through treatment simulations, predicting equipment failures, and serving as valuable tools for education and training. Additionally, this technology plays a crucial role in research and development, optimizing healthcare systems, and designing personalized medicine plans. Digital twins find diverse healthcare applications, including clinical trials, remote patient monitoring, customized medicine, surgery planning, epidemic management, prosthetics and implants, medical device development, and hospital operations.
Nevertheless, adopting digital twins in healthcare poses challenges, including data security and privacy concerns, data accuracy and completeness, interoperability issues, and the necessity for scalable implementation. Despite these challenges, the future of healthcare appears promising, driven by the revolutionary potential brought about by digital twins.
Aerospace
Digital twins, a transformative technology in aerospace, harness big data to revolutionize processes and drive innovation in research and development. Serving as virtual models reflecting physical equipment, these twins incorporate multiple sensors that relay performance data, enabling businesses to conduct simulations for studying and enhancing efficiency, impact analysis, and performance monitoring.
The aerospace industry reaps key benefits from digital twins, including more effective equipment development, improved efficiency, and predictive maintenance. These digital replicas extend the life of parts, enhance the next generation of machinery, and aid engineering in various conditions. Boeing, for instance, achieved a remarkable 40% improvement in the first-time quality of parts through digital twin implementation. Companies such as Rolls-Royce, Boeing, and Airbus are prominent companies that use digital twins in the aviation ecosystem. Digital twin technology has transformed the aviation industry, improving efficiency, sustainability, safety, and overall performance. As the industry continues to embrace this innovation, digital twins are set to play a critical role in shaping the future of aviation.
Startup ecosystem
Digital twin companies are gaining traction, and funding for these startups is increasing. Leucine, a New York City-based startup, raised $7 million in series A funding led by Ecolab to scale up its AI-generated digital twin platform to help drug development. Twin Health, a digital metabolic care startup, raised $50 million in funding led by Temasek to scale the availability of its Whole Body Digital Twin, an AI-backed model that aims to provide individualized nutrition. Neara, an energy sector digital twin startup, raised $15.25 million in an extension of last year’s $20 million series B funding round to gain offshore clients and expand in Europe. Forward Networks, a startup that has built digital twin modeling software geared towards modeling, managing, and securing complex enterprise networks, raised $50 million in series D funding led by MSD Partners. It has been estimated that the total funding raised by digital twin companies is more than USD 3.8 billion, with over 250 funded companies.
Below are the brief funding details of start-up in the ecosystem of Digital Twin
S.No. |
Company Name |
Funding (USD Million) |
Funding Date |
1 |
Twin Health |
50.0 |
Dec-23 |
2 |
Leucine |
7.0 |
Oct-23 |
3 |
Neara |
15.3 |
Oct-23 |
4 |
PrediSurge |
7.0 |
Sep-23 |
5 |
Peaxy |
12.0 |
Jul-23 |
7 |
VEERUM |
9.3 |
May-23 |
8 |
Intangles |
10.0 |
Feb-23 |
9 |
Prevu3D |
10.0 |
Feb-23 |
10 |
RIIICO |
1.5 |
Feb-23 |
11 |
Slingshot Simulations |
3.8 |
Jan-23 |
12 |
Forward Networks |
50.0 |
Jan-23 |
13 |
Worlds Enterprises Inc. |
16.5 |
May-22 |
16 |
vHive |
25.0 |
Apr-22 |
Note: The information provided above represents and does not encompass the entire ecosystem of Digital Twins.
Key contributors to the advancement of digital twin technology may encompass major players in the digital twin market, including General Electric, Microsoft, Siemens, Amazon Web Services, ANSYS, Dassault Systèmes, PTC, and Robert Bosch.
About Digital Twin Market study Published by Marketsandmarkets
The digital twin market report from MarketsandMarkets will serve as a valuable resource for the client to comprehend the present scenario and future possibilities within the digital twin market. It furnishes an extensive competitive analysis featuring key players, their brief profiles, and recent advancements, offering insightful perspectives into market dynamics and the competitive landscape. Additionally, the report provides historical data, forecast figures, and a comprehensive company analysis dashboard, empowering the client to identify lucrative growth opportunities and make well-informed decisions. The Digital Twin Market is expected to grow at a CAGR of more than 60% from 2023 to 2028 and may reach an estimated size of more than USD 100 Billion by 2028.
