Meet Microsoft Orca, a 13 Billion Parameter Small AI Model
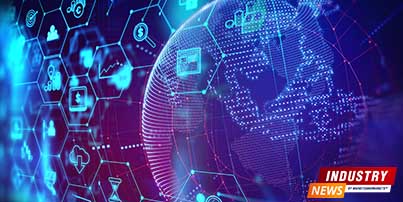
This News Covers
- Is Microsoft's Orca free? Where can I get Orca?
- What is a large foundation model?
- Orca Vs ChatGPT - what are the differences? - Pricing, scale and functionality wise.
- What will it mean when Orca is made open to public? - Will public be able to use it like ChatGPT? How can one build own AI model using Orca? What are other possible usages by inudstries, researchers, scholars?
- What are small AI models vs large AI models? Describe their features, limitations, GPU requirements to run a model, training requirements and more.
- How will Azure change with this partnership with Orca?
- What is BBH benchmark?
- What are ChatGPT's different products and what are they used for?
Microsoft's Orca AI is making waves in the field of artificial intelligence with its breakthrough capabilities. This 13-billion parameter small AI model is designed to imitate the complex reasoning process of large foundation models (LFMs) like GPT-4. By learning from rich signals, including explanation traces and step-by-step thought processes, Orca bridges the gap between small models and their larger counterparts. With its progressive learning approach and access to diverse imitation data, Orca surpasses existing instruction-tuned models and exhibits impressive performance on complex reasoning benchmarks. Microsoft's Orca represents a significant advancement in AI capabilities, promising enhanced reasoning and comprehension skills in a smaller package.
MarketsandMarkets welcomes this new era of AI with Microsoft's Orca, a 13-billion parameter small AI model that redefines possibilities and our editors share their views.
Is Microsoft's Orca free? Where can I get Orca?
Microsoft's Orca AI model is indeed intended to be free. It's part of Microsoft's research to advance the field of artificial intelligence, and it is designed for non-commercial research purposes. However, does not specify exactly where you can download or access Orca. Since Microsoft is the creator of Orca, it's likely that they would provide access to it through their own platforms, such as GitHub or their official website, once it becomes open-source. The excerpt also refers to the MS MARCO and ORCAS datasets, which are also provided by Microsoft for non-commercial research purposes. These are separate entities from the Orca model and are specific datasets used in AI research.
The key advantages and features of Microsoft's Orca AI model include:
- Imitating Reasoning Processes of LFMs: Orca is capable of learning complex explanation traces and step-by-step thought processes from GPT-4, a Large Foundation Model (LFM). This allows Orca to understand and replicate the reasoning processes used by these more complex models.
- Enhanced Learning via Explanation Traces: The incorporation of detailed responses, or explanation traces, provides valuable guidance for the model, improving its reasoning and comprehension skills.
- Use of Diverse Task Sampling: The researchers employed tasks from the Flan 2022 Collection to ensure a varied mix of challenges. This diverse and rich training set allowed Orca to learn to tackle a wide range of tasks effectively.
- Superior Performance on Benchmarks: Orca showed significant improvements over other models, such as Vicuna-13B, on the BigBench Hard (BBH) benchmark. It also demonstrated competitive performance on academic exams in zero-shot settings.
- Potential for Real-World Applications: Orca's success on academic exams and its superior performance compared to other models suggest potential for various real-world applications.
- Promising Approach for Future Research: Orca's successful application of learning from step-by-step explanations opens up exciting prospects for future research in AI and natural language processing. By refining the learning process from complex explanation traces, researchers may be able to enhance model performance across various tasks.
In summary, Orca represents a significant advancement in AI capabilities due to its ability to learn explanation traces from more complex models and its performance on various tasks and benchmarks. This approach could potentially drive further progress in the field of AI and natural language processing.
What is a large foundation model?
A Large Foundation Model (LFM) in the context of artificial intelligence, particularly in natural language processing and machine learning, refers to a class of models that are characterized by a large number of parameters and are usually pretrained on a large amount of data.
LFMs like GPT-4 or ChatGPT are capable of a wide range of tasks without needing task-specific fine-tuning because of their extensive training on diverse datasets. They exhibit remarkable zero-shot learning capabilities, which means they can generalize knowledge to perform tasks they were not explicitly trained to do.
The parameters of these models can be fine-tuned to suit a specific task or application. However, a key challenge is the supervision of these models' behavior, given their complexity and size. This has led to research into models like Orca, which are capable of learning complex explanation traces and step-by-step thought processes from LFMs.
The strength of LFMs lies in their ability to generalize across a wide range of tasks, handle complex queries, and generate highly sophisticated responses. They are commonly used in applications like conversational AI, content generation, and many other tasks that require understanding and generating human language. As these models continue to evolve, they're expected to play a critical role in the development of artificial intelligence and natural language processing systems.
Orca Vs ChatGPT - what are the differences? - Pricing, scale and functionality wise.
Orca and ChatGPT, while both being AI models capable of natural language processing, differ in their design, functionality, and the learning approach they use:
- Design and Scale: Orca is a 13-billion parameter model developed by Microsoft, learning to imitate the reasoning process of Large Foundation Models (LFMs) like GPT-4. The goal of Orca is to enhance the performance of existing state-of-the-art instruction-tuned models. ChatGPT, developed by OpenAI, is a variant of GPT-3, another LFM, but it has been specifically fine-tuned for generating conversational responses. Its parameters and scaling can vary with versions, but it's typically a lot smaller than Orca, with GPT-3 itself being a 175-billion parameter model.
- Learning Approach: Orca uses a novel learning approach where it learns from rich signals from GPT-4, including explanation traces, step-by-step thought processes, and other complex instructions. These explanation traces and complex instructions equip Orca with improved reasoning and comprehension skills, allowing it to better understand the reasoning process used by its teacher models. ChatGPT, on the other hand, was trained using Reinforcement Learning from Human Feedback (RLHF). It was initially trained using a dataset created from human interactions on the OpenAI Playground and was subsequently fine-tuned using Proximal Policy Optimization.
- Functionality: Both models have advanced language generation capabilities and can be used for a wide variety of natural language processing tasks. Orca, however, has been designed specifically to imitate the reasoning process of LFMs, while ChatGPT has been fine-tuned specifically for generating human-like text in a conversational context.
- Pricing: OpenAI had moved to a paid usage model for ChatGPT via the ChatGPT Plus subscription, with free access still available but with some limitations. For Microsoft's Orca, as of the sources you provided, there is no explicit information on the pricing or if it will be made publicly available.
- Unique Features: ChatGPT, like other models from OpenAI, has a unique feature set defined by its training and design. This includes:
- Tokens: ChatGPT operates at the token level, and the number of tokens in an API call affects the cost, response time, and whether the call works at all depending on the maximum limit. A token can be as short as one character or as long as one word, like "a" or "apple". Temperature: This parameter helps control the randomness of the model's output. A higher value (closer to 1) makes the output more diverse but potentially less coherent, while a lower value (closer to 0) makes the output more deterministic and focused. Max tokens: This is a guardrail to control the length of the generated text. If the model generates too much text, it might get cut-off, potentially leading to outputs that don't make sense.
The development and usage of AI models like Orca and ChatGPT are continually evolving. For the latest updates, you should refer to official resources from Microsoft and OpenAI.
What will it mean when Orca is made open to public? - Will public be able to use it like ChatGPT? How can one build own AI model using Orca? What are other possible usages by inudstries, researchers, scholars?
The public availability of Orca would provide users, developers, researchers, and organizations with access to a powerful AI model capable of imitating the reasoning process of Large Foundation Models (LFMs). It's important to note that the specific capabilities and usage possibilities would depend on the exact nature of the public release, as this could range from an open-source release of the model itself to a cloud-based API service, similar to what OpenAI has done with ChatGPT.
If made available in a manner similar to ChatGPT, users might be able to directly interact with Orca, use it for conversational AI, information extraction, or other natural language processing tasks. This could be via a user interface, or through an API, which could be integrated into applications, services, or research projects.
If Orca were released with its training code and methodology, it would offer researchers and developers the opportunity to fine-tune the model on specific tasks or datasets, thereby creating custom AI solutions. However, keep in mind that training or fine-tuning such a large model requires significant computational resources and expertise in machine learning and AI.
In terms of use-cases, the possibilities are extensive:
- Industries: Various industries could use Orca to power their AI applications. For instance, in customer service, Orca could be used to build more sophisticated chatbots capable of more complex reasoning. In healthcare, it could be used to provide explanations for diagnostic AI systems. In law or finance, it could help analyze and summarize complex documents.
- Researchers: Researchers in the field of AI and machine learning could use Orca as a benchmark to compare their models. They could also build upon the learning methods used in Orca to create new, more efficient models.
- Scholars: Scholars in social sciences, humanities, and other fields could use Orca to analyze large volumes of text data, uncover patterns, generate insights, or even write academic papers.
- Education: In educational settings, Orca could be used to create advanced tutoring systems that can provide detailed explanations to complex problems.
What are small AI models vs large AI models? Describe their features, limitations, GPU requirements to run a model, training requirements and more.
The distinction between small and large AI models typically refers to the number of parameters in the model. These parameters are the parts of the model that are learned from historical training data.
Small AI Models:
Features:
- Small models generally have fewer parameters, typically in the range of millions or tens of millions.
- They are simpler and easier to train.
- They can often run on commodity hardware and require less computational resources for both training and inference.
- They might be more suitable for tasks where data is limited, or simplicity and interpretability are required.
Limitations:
- Due to their simplicity, small models may lack the capacity to learn and generalize complex tasks.
- They are less capable of leveraging large amounts of data and might not perform as well as large models on tasks involving complex reasoning or comprehension.
GPU Requirements & Training:
- Small models can often be trained on a single GPU or a small cluster of GPUs. The exact requirements depend on the model architecture and the dataset size.
- The training time will be much less than that of larger models, and they might be trained using standard machine learning frameworks like TensorFlow or PyTorch without requiring specialized hardware or software.
Large AI Models:
Features:
- Large models have many more parameters, ranging from hundreds of millions to billions or even trillions.
- They can leverage large-scale data and exhibit powerful generalization capabilities when trained on diverse datasets.
- They are capable of learning more complex patterns and can handle more sophisticated tasks, including natural language understanding, image recognition, and complex reasoning.
Limitations:
- They require significant computational resources to train and may need specialized hardware or software.
- The high resource requirements can lead to a high environmental impact and limit accessibility to organizations with significant resources.
- Their complexity can make them harder to interpret and control, and they might require specialized techniques to fine-tune or adapt to specific tasks.
GPU Requirements & Training:
- Training large models often requires a cluster of high-end GPUs or specialized hardware like Google's TPU. The exact requirements will depend on the model's size and the training data.
- Training can take weeks or months even on high-end hardware, and typically involves large-scale distributed computing. Optimized software frameworks for distributed training, like Google's TPU Pods or Nvidia's NCCL, are often used.
How will Azure change with this partnership with Orca?
- Enhanced AI Services: With Orca, Azure could possibly improve its AI offerings by leveraging the capabilities of this advanced AI model. It may result in more efficient, accurate, and sophisticated AI services for natural language processing, data analysis, predictive modeling, etc.
- Better Tools for Developers: Azure could offer developers better tools and APIs for leveraging Orca, which could simplify the process of incorporating advanced AI capabilities into their applications.
- AI Training and Tuning Services: Azure might provide services for training and fine-tuning Orca on user-specific datasets, which could help organizations customize Orca's capabilities to their specific needs.
- Expanded Research Opportunities: For researchers, Azure may offer more extensive resources and tools for AI research. This could attract more academic and industry researchers to the platform, fostering a vibrant research community around it.
- Competitive Edge: Incorporating advanced AI models like Orca can provide Azure with a competitive edge over other cloud services, making it a more attractive choice for organizations that require sophisticated AI capabilities.
- Educational Resources: Azure might also develop educational resources, courses, and certifications focused on using and understanding Orca, thereby encouraging skill development in the community.
- AI Ethics and Governance: Given the potential of large AI models to impact society, Azure might also strengthen its focus on AI ethics, responsible AI use, and governance.
What is BBH benchmark?
The BBH (BigBench Hard) benchmark is a specific evaluation measure used to assess the performance and capabilities of AI models, particularly in the context of instruction-tuned models and reasoning tasks. It is designed to evaluate the models' abilities in complex reasoning and comprehension tasks.
The BBH benchmark is characterized by challenging prompts or queries that require advanced reasoning skills to generate accurate and comprehensive responses. These prompts often involve complex scenarios, multiple steps, and require deep understanding and logical reasoning to provide appropriate answers.
By evaluating AI models on the BBH benchmark, researchers and developers can assess the models' performance and compare their capabilities in handling complex reasoning tasks. It serves as a way to gauge how well the models can imitate the reasoning process of large foundation models (LFMs) and to measure their advancement in instruction-tuned models.
In the context of the discussion about Orca, the reference to Orca's performance on the BBH benchmark indicates that it has shown significant improvement and competitiveness in tackling challenging reasoning tasks when compared to other instruction-tuned models like Vicuna-13B.
What are ChatGPT's different products and what are they used for?
ChatGPT, developed by OpenAI, is a family of language models that excel in generating human-like text responses based on given prompts. While the specific product lineup may evolve over time, as of my knowledge cutoff in September 2021, there are a few notable ChatGPT variants:
- GPT-3: GPT-3 (Generative Pre-trained Transformer 3) is one of the earlier versions of ChatGPT. It consists of a massive neural network with 175 billion parameters, making it one of the largest language models at the time of its release. GPT-3 offers impressive language generation capabilities and is widely used for various tasks, including chatbots, content generation, and text completion.
- GPT-4: While not explicitly mentioned in the information you provided, GPT-4 is referenced as the teacher model that guides Orca's learning process. GPT-4 represents a more advanced version of ChatGPT, building upon the successes and advancements of its predecessors. It is likely that GPT-4 provides improved reasoning abilities and generates high-quality responses to assist in Orca's training.
It's worth noting that the specific product offerings and capabilities of ChatGPT may have expanded or evolved since my last knowledge update. For the most up-to-date information, I recommend referring to OpenAI's official documentation or announcements regarding ChatGPT and its different products.
References
GET AHEAD
Top Research Reports to Fuel Your Industry Knowledge- Composite AI Market by Offering (Software, Hardware, Services), Technique (Data Processing, Pattern Recognition), Application (Product Design & Development, Customer Service), Vertical and Region - Global Forecast to 2028
- Autonomous AI and Autonomous Agents Market by Offering (Hardware, Software (Type (Computational Agents, Robotic Agents)) and Services), Technology (ML, NLP, Context Awareness, Computer Vision), Vertical and Region - Global Forecast to 2028
- Embedded AI Market by Offering (Hardware, Software, Services), Data Type (Numerical Data, Categorical Data, Image & Video Data), Vertical (Automotive, Manufacturing, Healthcare & Life Sciences, Telecom), and Region - Global Forecast to 2028
Editor's Pick
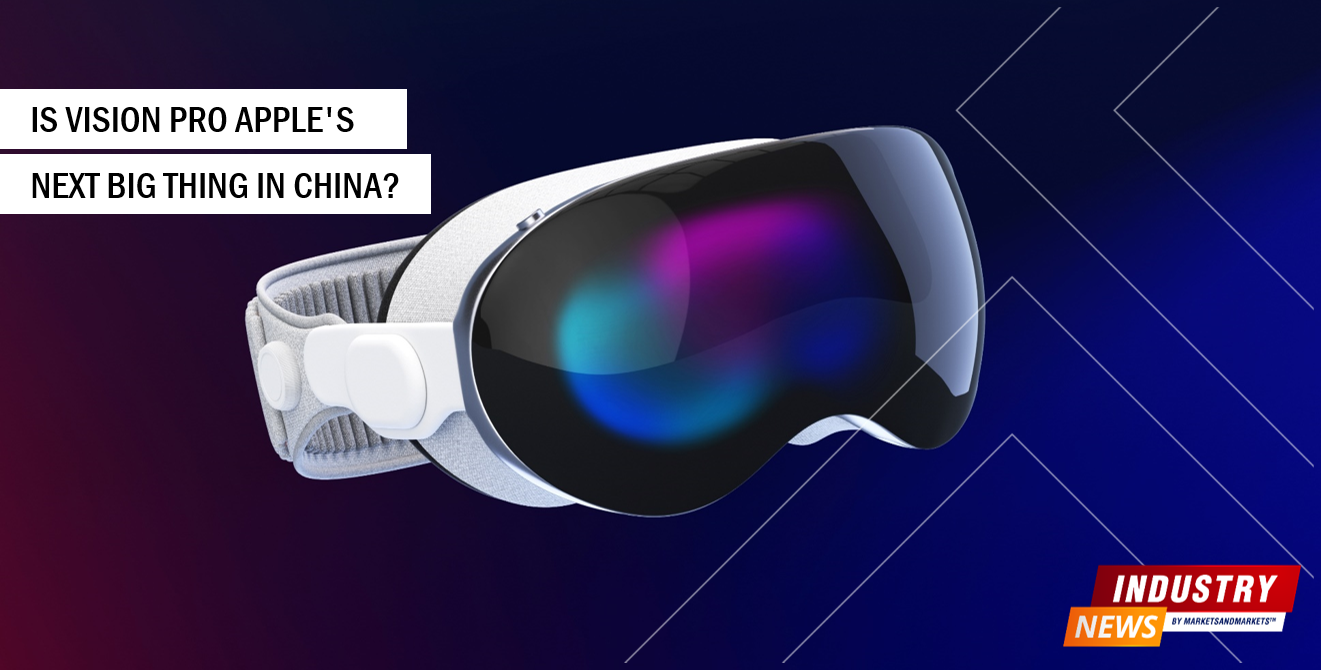
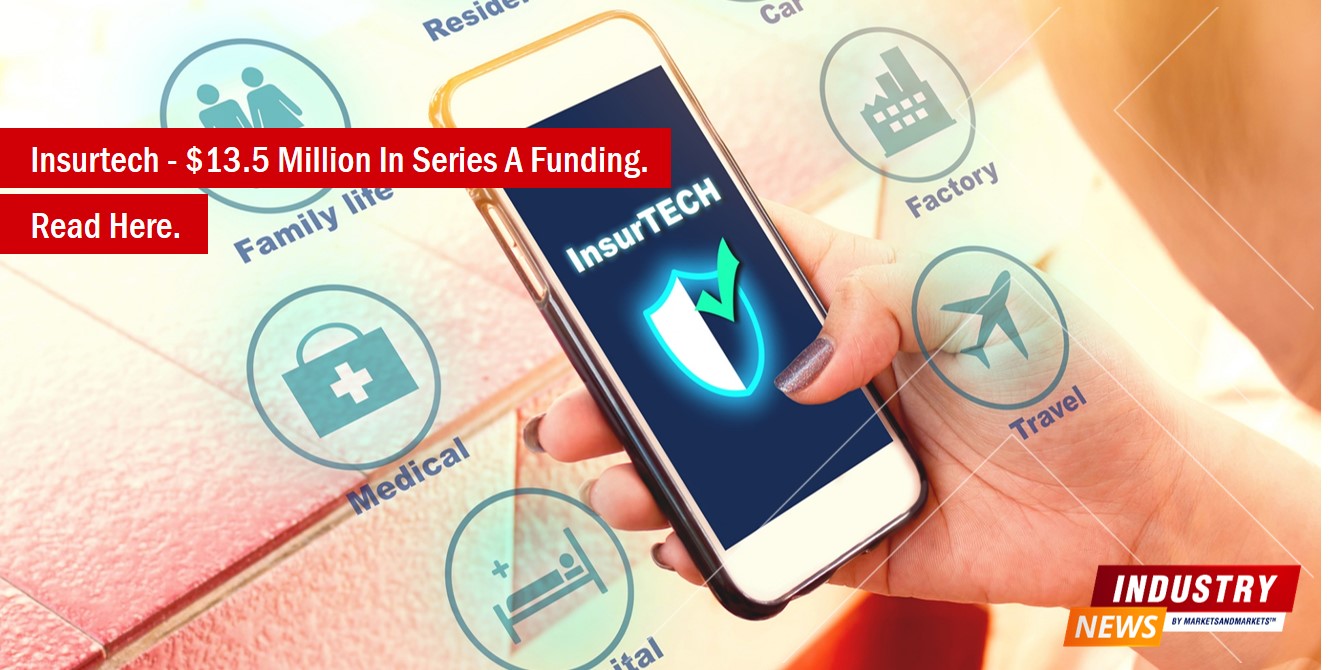
Information and Communication Technology
Insurtech Funding News - Coverdash raises USD 13.5 Million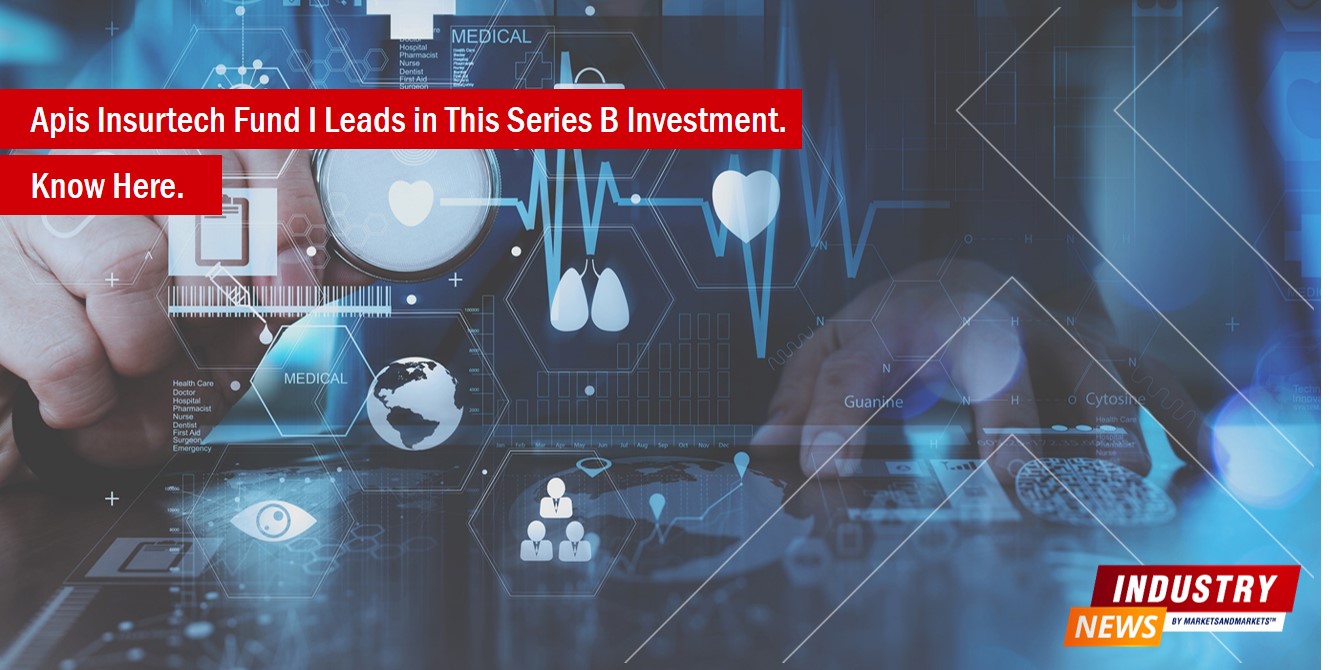
PODCASTS
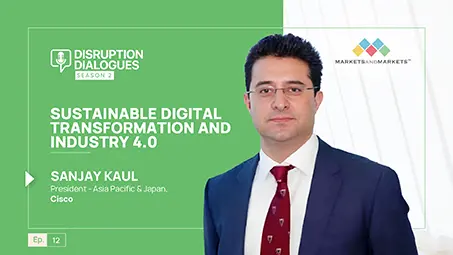
Sustainable Digital Transformation & Industry 4.0
Sanjay Kaul, President-Asia Pacific & Japan, Cisco, and host Aashish Mehra, Chief Research Officer, MarketsandMarkets, in conversation on unraveling 'Sustainable Digital Transformation and Industry 4.0'
11 July 2023|S2E12|Listen Now
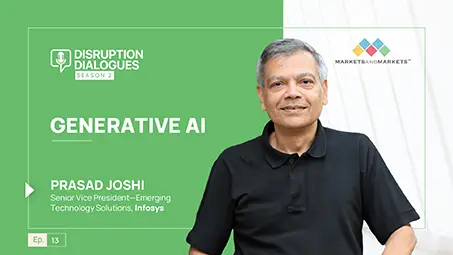
Generative AI
Prasad Joshi, Senior Vice President-Emerging Technology Solutions, Infosys, and host, Vinod Chikkareddy, CCO, MarketsandMarkets, in exploring the recent advances in AI and the generative AI space.
7 Nov 2023|S2E13|Listen Now
Embedded AI Market


Download Whitepaper
Orca AI, a 13-billion parameter model, pushes the boundaries of AI.
Orca's free availability for non-commercial research marks a significant step in AI.
